
What causes the heterogeneity of treatment effect?
1 Heterogeneous Treatment Effects Same treatment may affect different individuals differently Conditional Average Treatment Effect (CATE) ˝(x) = E(Y i(1) Y i(0) jX i = x) where x 2X Individualized treatment rule f : X! f 0;1g We can never identify an individual causal effect ˝ i = Y i(1) Y i(0) Individualized treatment rule depends on the choice of X i
Does the workhorse regression work for heterogeneous treatment effects?
researchers interested in heterogeneous treatment effects are likely to encounter the problem of multiple comparisons: for example, when numerous subgroup analyses are conducted, the probability that at least one result looks statistically significant at the 5 percent level may be considerably greater than 5 percent even when the treatment has no …
Why is it difficult to study treatment effects in observational studies?
Regressions that control for confounding factors are the workhorse of evaluation research. When treatment effects are heterogeneous, however, the workhorse regression leads to estimated treatment effects that lack behavioral interpretations even when the selection on observables assumption holds. Regressions that use propensity scores as weights and regressions based …
What is the null hypothesis of a constant treatment effect?
A relatively recent literature has tried to study heterogeneous treatment effects in which these things vary across individuals ... the full distribution of treatment effects is not identified. For example suppose Ti is being pregnant and ment are never pregnant, we could never how to identify E(Income jPregnant, Male)

How do you test for heterogeneous treatment effects?
To implement the test, first use the experimental data to estimate the average treatment effect (ATE) and the difference in variances Var(Yi(1))−Var(Yi(0)). Next, create a full hypothetical schedule of potential outcomes assuming that the true treatment effect is constant and equal to the estimated ATE.
What is homogeneous treatment effect?
A homogeneous treatment effects model. The magnitude and direction of the treatment effect is the same for all patients, regardless of any other patient characteristics. Models that allow the treatment effect to be different for different individuals are referred to as heterogeneous treatment effect models.May 21, 2016
What are treatment effects in research?
A 'treatment effect' is the average causal effect of a binary (0–1) variable on an outcome variable of scientific or policy interest.
What is a differential treatment effect?
In this context, differential treatment effects include not only quantitative variations in the magnitude of treatment effects occurring across subgroups, but also qualitative differences in treatment effects (that is, a given treatment is significantly better than an alternative one in some groups of individuals, and ...Jun 20, 2017
What is heterogeneous treatment?
Heterogeneity of treatment effect (HTE) is the nonrandom, explainable variability in the direction and magnitude of treatment effects for individuals within a population.
What is called heterogeneous?
: consisting of dissimilar or diverse ingredients or constituents : mixed an ethnically heterogeneous population.
What is treatment on the treated effect?
the treatment effect on the treated group equals the treatment effect on the control group (layman terms: people in the control group would do as good as the treatment group if they were treated).Oct 25, 2017
What is treatment on treated?
ITT (Intent to Treat) = People made eligible for treatment / intervention. TOT (Treatment on the Treated) = People who actually took the. treatment / intervention.
What is the sample average treatment effect?
In contrast, the sample average treatment effect (SATE) is the mean difference in the counterfactual outcomes for the study units. The sample parameter is easily interpretable and arguably the most relevant when the study units are not sampled from some specific super-population of interest.Apr 18, 2016
What is differential effects in psychology?
The differential outcomes effect is a theory in behaviorism, a branch of psychology, that shows that a positive effect on accuracy occurs in discrimination learning between different stimuli when unique rewards are paired with each individual stimulus.
What is the curse of dimensionality?
The curse of dimensionality, is a causal inference paradox describing situations in which leveraging more information in an analysis can decrease the accuracy of a CATE estimate. In order to avoid this curse, an analyst will need to be clever about how they select the confounding variables they condition on when attempting to calculate an unbiased CATE estimation. In academic fields, such as economics, medicine, and epidemiology, analysts often rely on underlying theoretical concepts to inform the specification of a smaller causal graph and more easily estimate causal effects.
How to estimate conditional average treatment effects?
One way an analyst can estimate conditional average treatment effects is subclassification, which splits observed individuals into subclassifications along variation in their respective values of confounding variables. For example, if an analyst wishes to understand the effect of their treatments on different genders, they may split their population of observed individuals by gender, and estimate causal effects within each defined gender. Once observed individuals have been split into subclassifications, an analyst can estimate causal effects within these groups by leveraging the simple difference in mean outcomes (SDO) calculation discussed in my post covering average treatment effect estimation. As the name suggests, leveraging the SDO requires an analyst to calculate the difference in the mean outcomes within each group with value#N#≈ z#N#color {#7A28CB} approx z ≈ z in order to estimate#N#τ ( z)#N#color {#7A28CB} tau (z) τ (z). An analyst can use this to estimate CATE as a result of a useful theorem from probability theory#N#E [ A − B] = E [ A] − E [ B]#N#E [A - B] = E [A] - E [B] E[A−B]= E[A]−E[B], and thus:
What is the figure 5?
In this visualization, similar observed individuals which are exposed and unexposed to an ad are matched, and translated to the matched samples chart in order to measure the difference in their outcomes. Within the three youngest matched samples both exposed and unexposed individuals did not purchase a flight, and from this information I can infer that I should target consumers older than 30 with my advertising campaign.
What is heterogeneous treatment effect estimation?
One of the most fruitful applications of heterogeneous treatment effect estimation is within the analytics of automated bidding systems for digital advertisements. Many businesses using digital advertising to expand their customer base use automated bidding systems to purchase ads programmatically on a digital advertisement platform, such as Google Ads. These platforms provide markets with an option to automatically bid for the opportunity to show an ad to a particular user, given a provided set of their characteristics. This purchasing model is quite different then traditional advertising marketplaces, such as those used for television, billboard, and newspaper ads. In those marketplaces, a marketer pays a lump sum to purchase an advertising opportunity accessing a particular swath of the population, (ESPN fans, Highway 101 commuters, New York Times readers) and is only really concerned with how many consumers purchase their product once they are exposed to the purchased ad. On the other hand, when a marketer designs an automated bidding system to interact with digital advertising platforms, they can purchase advertising opportunities which target specific individuals most likely to subsequently convert (which means to visit the marketer’s site and take a revenue-generating action). In order to maximize the resultant profits of an advertising campaign, marketers often try to estimate the heterogeneous treatment effects of their campaigns to ensure they only purchase expensive digital advertisements that target consumers most likely to be influenced.
Abstract
Heterogeneous treatment effect (HTE) analysis focuses on examining varying treatment effects for individuals or subgroups in a population. For example, an HTE-informed understanding can critically guide physicians to individualize the medical treatment for a certain disease.
INTRODUCTION
Treatment effect refers to the causal effect of a treatment or intervention (e.g., administering an anticancer drug) on an outcome of interest (e.g., health or disease progression of the patient) based on the counterfactuals (e.g., difference in outcomes with/without using the drug).
METHODS
In this section, after describing the basic principle of treatment effect, we introduce the concept of HTE and graphically illustrate the differences between no treatment heterogeneity and HTE.
RESULTS
In this section, a case example with nonlinear HTE was simulated to demonstrate HTE analysis conducted by causal forest and the two-step method. By stipulating Equation ( 1 ), the example case was simulated based on the model , where , and heterogeneity covariates and have interactions with the treatment ( T) in a nonlinear form, ( ).
DISCUSSION
This study introduced an ML-based HTE analysis and presented a systematic evaluation of HTE analysis methods. HTE analysis demonstrated its ability to address questions on the individualized counterfactual treatment effects.
CONCLUSION
Given its resilience in handling complex data (e.g., nonlinear and/or high-dimensional data), the causal forest HTE method, an ML approach derived from a random forest algorithm, provides a unique opportunity for scientists to assess and predict heterogeneity for treatment effect for real-world applications.
DISCLAIMER
The opinions expressed in this manuscript are those of the authors and should not be interpreted as the position of the US Food and Drug Administration.
Access Options
You can be signed in via any or all of the methods shown below at the same time.
Abstract
Regressions that control for confounding factors are the workhorse of evaluation research. When treatment effects are heterogeneous, however, the workhorse regression leads to estimated treatment effects that lack behavioral interpretations even when the selection on observables assumption holds.
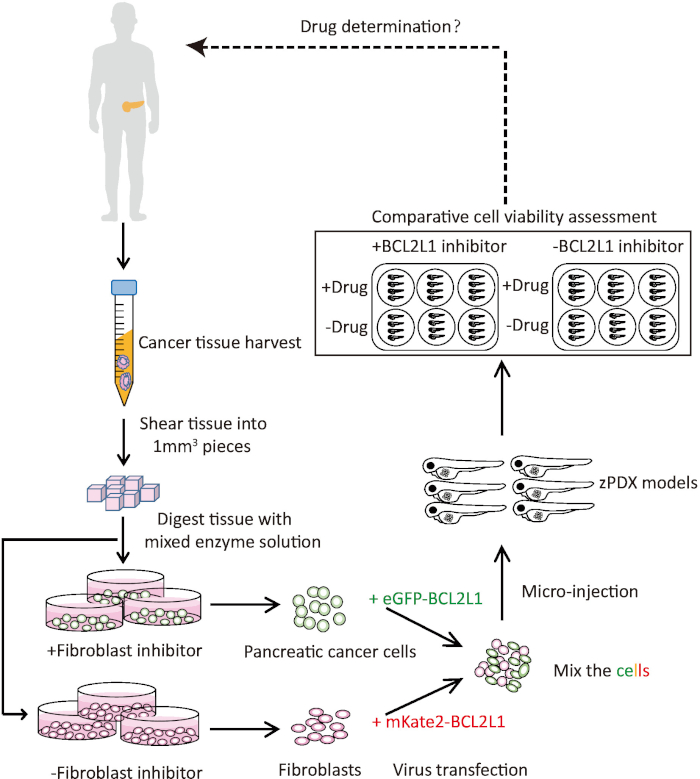