
Outlier Treatment is One of the important part of data pre processing is the handling outlier. If your data contains outliers that affect our result which will depend on the data. So to remove these outliers from data Outlier Treatment is used.
Full Answer
How to treat outliers?
Treating outliers: A subjective task. Similar to not detecting outliers at all, handling outliers can bear the risk of having a substantial impact on the outcome of an analysis or machine learning model.
What to do with outliers?
MeSH terms
- Child
- Data Interpretation, Statistical
- England
- Hospital Mortality*
- Humans
- Intensive Care Units, Pediatric / standards*
- Intensive Care Units, Pediatric / statistics & numerical data
- Outcome Assessment, Health Care / statistics & numerical data*
- Risk Adjustment
- Wales
How to solve outliers?
This method has several shortcomings :
- The mean and standard deviation are strongly affected by outliers.
- It assumes that the distribution is normal (outliers included)
- It does not detect outliers in small samples
How do you evaluate outliers?
To run outlier root cause analysis:
- In the TCM dialog, go to the Build Options tab and then click Series to Display in the Select an item list. ...
- Move KPI_19 to the Fields to display list.
- Click Output options in the Select an item list on the Options tab. ...
- Deselect Overall model system, Same as for targets, R square, and Series transformations.
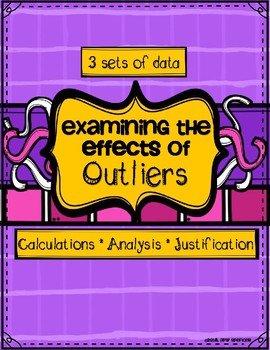
What are outlier treatments?
In this article, we have seen 3 different methods for dealing with outliers: the univariate method, the multivariate method and the Minkowski error. These methods are complementary and, if our data set has many and difficult outliers, we might need to try them all.
Why do we do outlier treatment?
Outliers increase the variability in your data, which decreases statistical power. Consequently, excluding outliers can cause your results to become statistically significant.
What is outlier treatment in machine learning?
It is an abnormal observation during the Data Analysis stage, that data point lies far away from other values. An outlier is an observation that diverges from well-structured data. The root cause for the Outlier can be an error in measurement or data collection error. Quick ways to handling Outliers.
How do you identify and treat outliers?
A first and useful step in detecting univariate outliers is the visualization of a variables' distribution. Typically, when conducting an EDA, this needs to be done for all interesting variables of a data set individually. An easy way to visually summarize the distribution of a variable is the box plot.
What do outliers mean?
Definition of outliers. An outlier is an observation that lies an abnormal distance from other values in a random sample from a population. In a sense, this definition leaves it up to the analyst (or a consensus process) to decide what will be considered abnormal.
What causes an outlier?
There are three causes for outliers — data entry/An experiment measurement errors, sampling problems, and natural variation. An error can occur while experimenting/entering data.
How are outliers treated in regression?
in linear regression we can handle outlier using below steps:Using training data find best hyperplane or line that best fit.Find points which are far away from the line or hyperplane.pointer which is very far away from hyperplane remove them considering those point as an outlier. ... retrain the model.go to step one.
How does outlier affect machine learning?
Machine learning algorithms are sensitive to the range and distribution of attribute values. Data outliers can spoil and mislead the training process resulting in longer training times, less accurate models and ultimately poorer results.
How do you remove outliers in ML?
There are some techniques used to deal with outliers.Deleting observations.Transforming values.Imputation.Separately treating.Deleting observations. Sometimes it's best to completely remove those records from your dataset to stop them from skewing your analysis.
What is the outlier formula?
The outlier formula designates outliers based on an upper and lower boundary (you can think of these as cutoff points). Any value that is 1.5 x IQR greater than the third quartile is designated as an outlier and any value that is 1.5 x IQR less than the first quartile is also designated as an outlier.
Why is it important to understand outliers?
Understanding the outliers is critical in analyzing data for at least two aspects: The outliers may negatively bias the entire result of an analysis; the behavior of outliers may be precisely what is being sought. While working with outliers, many words can represent them depending on the context. Some other names are: Aberration, oddity, ...
What is an outlier in data analysis?
In other words, an outlier is a value that escapes normality and can (and probably will) cause anomalies in the results obtained through algorithms and analytical systems. There, they always need some degrees of attention. Understanding the outliers is critical in analyzing data for at least two aspects: The outliers may negatively bias the entire ...
How to identify which record is outlier?
The simplest way to find outliers in your data is to look directly at the data table or worksheet – the dataset, as data scientists call it. The case of the following table clearly exemplifies a typing error, that is, input of the data. The field of the individual’s age Antony Smith certainly does not represent the age of 470 years. Looking at the table it is possible to identify the outlier, but it is difficult to say which would be the correct age. There are several possibilities that can refer to the right age, such as: 47, 70 or even 40 years.
What is Aquarela Advanced Analytics?
Aquarela Analytics is Brazilian pioneering company and reference in the application of Artificial Intelligence in industry and large companies. With the Vortx platform and DCIM methodology, it serves important global customers such as Embraer (aerospace), Randon Group (automotive), Solar Br Coca-Cola (food), Hospital das Clínicas (health), NTS- Brazil (oil and gas), Votorantim (energy), among others.
How to identify outliers in statistics?
We have seen that it is imperative to pay attention to outliers because they can bias data analysis. But, in addition to identifying outliers we suggest some ways to better treat them: 1 Exclude the discrepant observations from the data sample: when the discrepant data is the result of an input error of the data, then it needs to be removed from the sample; 2 perform a separate analysis with only the outliers: this approach is useful when you want to investigate extreme cases, such as students who only get good grades, companies that make a profit even in times of crisis, fraud cases, among others. use clustering methods to find an approximation that corrects and gives a new value to the outliers data. 3 in cases of data input errors, instead of deleting and losing an entire row of records due to a single outlier observation, one solution is to use clustering algorithms that find the behavior of the observations closest to the given outlier and make inferences of which would be the best approximate value.
What percentage of data is outliers?
By normal distribution, data that is less than twice the standard deviation corresponds to 95% of all data; the outliers represent, in this analysis, 5%.
What are some words that represent outliers?
Some other names are: Aberration, oddity, deviation, anomaly, eccentric, nonconformist, exception, irregularity, dissent, original and so on. Here are some common situations in which outliers arise in data analysis and suggest best approaches on how to deal ...
What is an outlier in statistics?
An outlier is a data point in a data set that is distant from all other observation.
What is an outlier in a data set?
We find the z-score for each of the data point in the dataset and if the z-score is greater than 3 than we can classify that point as an outlier. Any point outside of 3 standard deviations would be an outlier.
Introduction To Outlier Treatment
Outlier Treatment is One of the important part of data pre processing is the handling outlier. If your data contains outliers that affect our result which will depend on the data. So to remove these outliers from data Outlier Treatment is used. First of all, need to understand what is outlies.
Interquartile Range ( IQR )
Interquartile Range ( IQR ) equally divides the distribution into four equal parts called quartiles. It takes data into account the most of the value lies in that region, It used a box plot to detect the outliers in data.
Identify the Outliers Using IQR Method
As per a rule of thumb, observations can be qualified as outliers when they lie more than 1.5 IQR below the first quartile or 1.5 IQR above the third quartile. Outliers are values that “lie outside” the other values.
Outlier Treatment using IQR in Python
Example:- In this example, we load data set from the seaborn library and apply IQR outliers treatment on the total bill column.
Outlier Treatment using Z-score in Python
Example:- In this example, we load data set from the seaborn library and apply Z-Score outliers treatment on the total bill column.
Conclusion
In this blog, you get the clear understanding of the Outlier treatment processes like IQR & Z Score and its working and their implementation on the data set. It will really helpful to Remove outliers from data and it also improve your knowledge of data preprocessing concepts.
Why do we delete outliers?
Deletion – We delete outlier values if it is due to data entry error, data preprocessing error or if outlier observations are very less in number. We can also trim at both ends to remove outliers from the dataset.
What is an outlier in statistics?
Outliers are nothing but data points that differ significantly from other observations. They are the points that lie outside the overall distribution of the dataset. Outliers, if not treated, can cause serious problems in statistical analyses.
What is an outlier in a scatter plot?
Scatter plots – Scatter plots often have a pattern. Although there is no special rule in scatter plots that tells us whether a point is outlier or not, but we call a data point an outlier if it doesn’t fit the pattern.
What is an outlier in a histogram?
Histograms – Outliers are often easy to spot in histograms. The data points that lie extremely away from the majority of data points are termed as outli ers.
Where are univariate outliers found?
Univariate Outliers – These outliers are found in the distribution of values in a single feature space.
Is KNN sensitive to outliers?
KNN (for small value of K, KNN is sensitive to outliers)
Do all machine learning models have outliers?
Not all machine learning models have issues with outliers. There are just few of them which are sensitive to outliers and are affected by them. Sensitivity of models to outliers is intuitive and can be understood by the statistics hidden behind each of these models. Such outlier sensitive machine learning models are listed below-
What is an outlier in statistics?
Outliers are observations with a unique combination of characteristics identifiable as distinctly different from the other observations. It is basically judged to be an unusually high or low value on variable or unique combinations of values across several variables that make the observations stand from others.
How does isolation forest work?
Isolation Forest isolates observations by randomly selecting a feature and then randomly selects a split value between the maximum and minimum values of that selected feature. It is an unsupervised algorithm and therefore it does not need labels to identify the outlier/anomaly. The PyOD Isolation Forest module is a wrapper of scikit learn Isolation Forest with more functionalities. Here I have worked on Superstore data and implemented Isolation Forest Algorithm to find outliers and calculate anomaly score among them.
What are the methods to deal with outliers?
In this article, we have seen 3 different methods for dealing with outliers: the univariate method, the multivariate method, and the Minkowski error . These methods are complementary and, if our data set has many severe outliers, we might need to try them all.
What is an outlier in statistics?
An outlier is a data point that is distant from other similar points. They may be due to variability in the measurement or may indicate experimental errors. If possible, outliers should be excluded from the data set . However, detecting that anomalous instances might be very difficult, and is not always possible.
What is an outlier in Tukey's method?
Tukey's method defines an outlier as those values of a variable that fall far from the central point, the median.
Do outliers need extreme values?
Outliers do not need to be extreme values. Indeed, as we have seen with Point B B, the univariate method does not always work well. The multivariate method tries to solve that by building a predictive model using all the data available and cleaning those instances with errors above a given value.
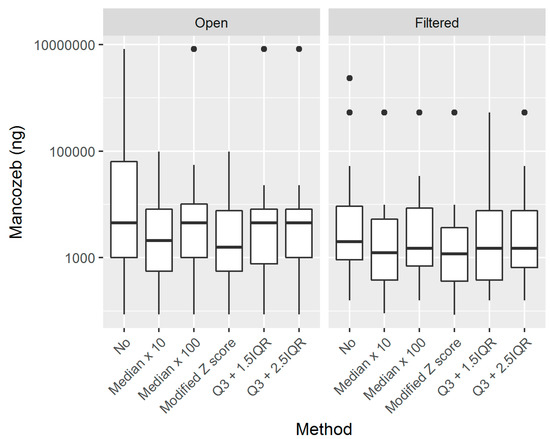