
principal econometric problem in the estimation of treatment effects is selection bias, which arises from the fact that treated individuals differ from the non-treated for reasons other than treatment status per se. Treatment effects can be estimated using social experiments, regression models, matching estimators, and instrumental variables.
Full Answer
What is econometric modelling?
A ‘treatment effect’ is the average causal effect of a binary (0–1) variable on an outcome variable of scientific or policy interest. The term ‘treatment effect’ originates in a medical literature concerned with the causal effects of binary, yes-or-no ‘treatments’, such as an experimental drug or a new surgical procedure.
What is the treatment effect for each individual?
A treatment e⁄ect is simply the causal e⁄ect ™treatment™(e.g. undergoing a training programme) on an outcome variable of interest (e.g. productivity at work). Typically the treatment variable is a binary (0-1) variable.
How do you calculate constant treatment eect?
Journal of Econometrics 67 (1995): 379-401. Oreopoulos, P. “Estimating Average and Local Average Treatment Effects of Education when Compulsory Schooling Laws Really Matter.” The American Economic Review 96, no. 1 (March 2006): 152-175.
How do you find the average treatment effect?
Treatment Effect Model. Secondly, we use a treatment effects model incorporating the results from the probit model in a general equation of efficiency to decompose the impact of liberalization on efficiency into a direct and an indirect impact. From: Emerging Markets Review, 2013. Download as PDF.
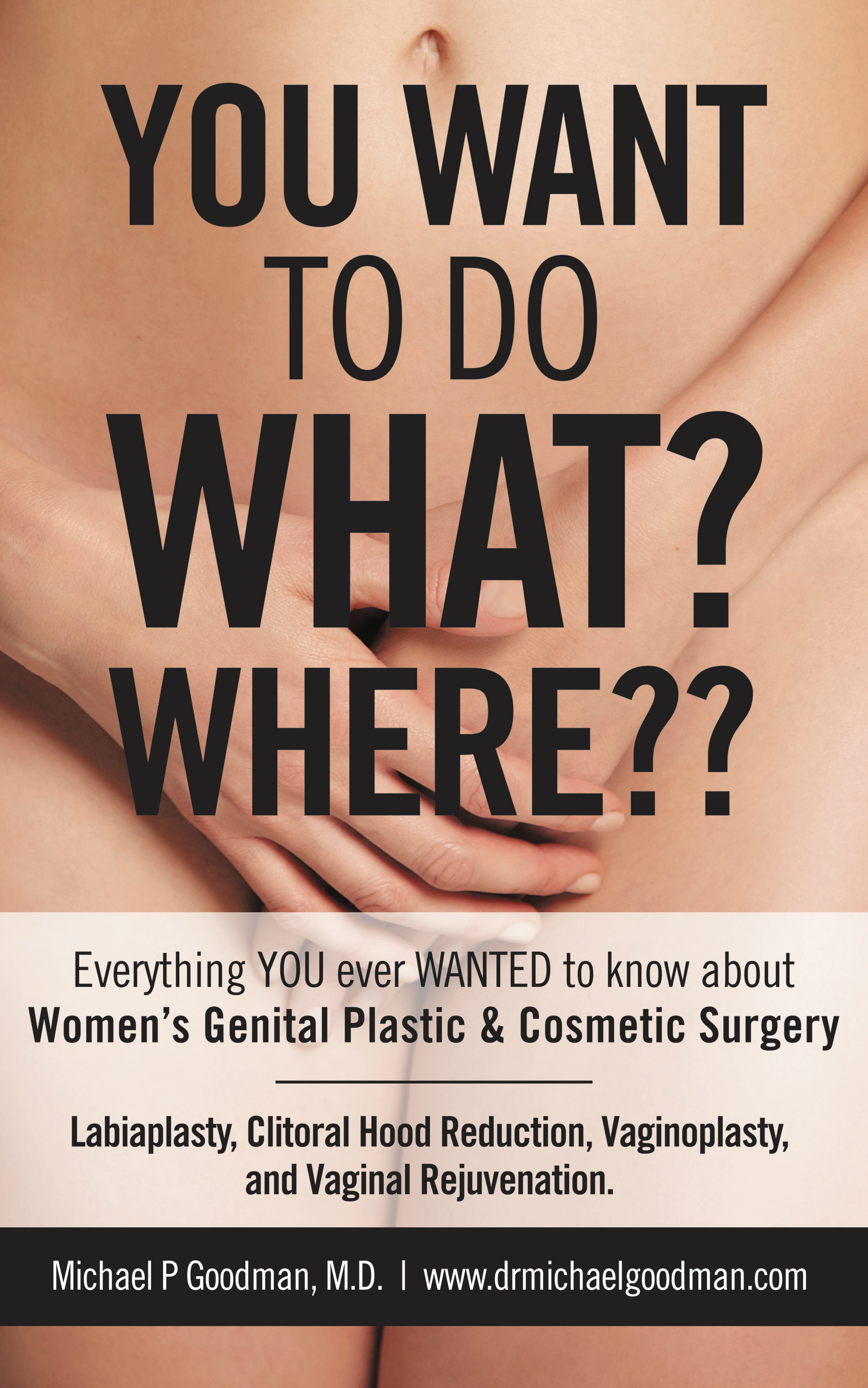
What is treatment in econometrics?
What is average treatment effect in econometrics?
How do you analyze treatment effects?
What is treatment on the treated effect?
What is treatment effect size?
What is treatment effect in psychology?
What is a large treatment effect?
An estimate of how large the treatment effect is, that is how well the intervention worked in the. experimental group in comparison to the control. group. The larger the effect size, the stronger are the.
How do you calculate average treatment effect?
What is heterogeneous treatment effects?
What is the difference between average treatment effect and average treatment effect on the treated?
What is treatment effect in Anova?
What is the difference between ATE and ATET?
Financial liberalization and stock markets efficiency: New evidence from emerging economies
In order to determine the impact of financial liberalization on informational efficiency, we use the treatment effects methodology developed by Maddala (1983)7. This methodology has been used widely in the literature, especially in the areas of economic growth and social economics ( Maeda, 2008; Ranciere et al., 2006 ).
Income shocks, coping strategies, and consumption smoothing: An application to Indonesian data
In this section we present the empirical strategy that we adopt to obtain a quantitative measure of the income reduction produced by the crop loss, and of the household's ability to recover from the shock. Several methodologies have been used to measure income shocks.
Background
There is a large literature in economics and public policy that seeks to evaluate the effect of various treatments and programs on outcomes of individuals.
Objectives
The purpose of this research is to develop a method to estimate treatment effects when treatment is endogenous, i.e. if is not random conditional on time and group fixed effects, and the sample size of the decision to treat is smaller than the sample size of outcomes.
How to calculate ATE?
Depending on the data and its underlying circumstances, many methods can be used to estimate the ATE. The most common ones are: 1 Natural experiments 2 Difference in differences 3 Regression discontinuity designs 4 Propensity score matching 5 Instrumental variables estimation
What is treatment in science?
Originating from early statistical analysis in the fields of agriculture and medicine, the term "treatment" is now applied, more generally, to other fields of natural and social science, especially psychology, political science, and economics such as, for example, the evaluation of the impact of public policies.
General definition
In the terminology from the potential outcomes framework,#N#Y i ( d i ) {\displaystyle Y_ {i} (d_ {i})}#N#denotes the potential outcome of subject#N#i {\displaystyle i}#N#, where#N#d i {\displaystyle d_ {i}}#N#is the binary indicator of subject#N#i {\displaystyle i}#N#’s treatment status.
Potential outcome framework
The causal effect of the treatment on subject#N#i {\displaystyle i}#N#is#N#Y i ( 1 ) − Y i ( 0 ) {\displaystyle Y_ {i} (1)-Y_ {i} (0)}#N#. However, we can never observe both#N#Y i ( 1 ) {\displaystyle Y_ {i} (1)}#N#and#N#Y i ( 0 ) {\displaystyle Y_ {i} (0)}#N#for the same subject.
Identification
The#N#I T T {\displaystyle ITT}#N#measures the average effect of experimental assignment on outcomes without accounting for the proportion of the group that was actually treated (i.e. average of those assigned to treatment minus the average of those assigned to control).
Application: hypothetical schedule of potential outcome under two-sided noncompliance
The table below lays out the hypothetical schedule of potential outcomes under two-sided noncompliance.
Others: LATE in instrumental variable framework
We can also think of LATE through an IV framework.
Generalizing LATE
The primary goal of running an experiment is to obtain causal leverage, and it does so by randomly assigning subjects to experimental conditions, which sets it apart from observational studies. In an experiment with perfect compliance, the average treatment effect can be obtained easily.
Further reading
Angrist, Joshua D.; Fernández-Val, Iván (2013). Advances in Economics and Econometrics. Cambridge University Press. pp. 401–434. doi: 10.1017/cbo9781139060035.012. ISBN 9781139060035.
What is a cate?
A CATE is an average treatment effect specific to a subgroup of subjects, where the subgroup is defined by subjects’ attributes (e.g., the ATE among female subjects) or attributes of the context in which the experiment occurs (e.g., the ATE among subjects at a specific site in a multi-site field experiment).
What is a cate in a treatment effect?
A CATE is an average treatment effect specific to a subgroup of subjects, where the subgroup is defined by subjects’ attributes (e.g., the ATE among female subjects) or attributes of the context in which the experiment occurs ( e.g., the ATE among subjects at a specific site in a multi-site field experiment).
How to test whether an interaction effect could have occurred by chance?
To test whether the estimated interaction effect could have occurred by chance, one can use randomization inference: First generate a full schedule of potential outcomes under the null hypothesis that the true treatment effect is constant and equal to the estimated ATE. Then simulate random assignment a large number of times and calculate how often the simulated estimate of the interaction effect is at least as large (in absolute value) as the actual estimate.
What is FWER in statistics?
Familywise error rate (FWER) control methods limit the probability of making at least one type I error given the number of tests conducted. Suppose one is testing K hypotheses, H 1, H 2, …, H K, and K 0 of the K hypotheses are true, where K 0 ≤ K. The familywise error rate is the probability that at least one of the K 0 true hypotheses is falsely rejected. The FWER increases in the number of hypotheses tested. FWER control methods adjust the p -values so that, for example, if we reject a hypothesis only when the adjusted p -value is less than 0.05, the FWER will not exceed 5 percent.
Why is machine learning useful?
Machine learning methods are useful to automate the search for systematic variation in treatment effects. These automated approaches are attractive because they minimize researchers’ use of ad hoc discretion in selecting and testing interactions, and are useful for conducting exploratory analyses.
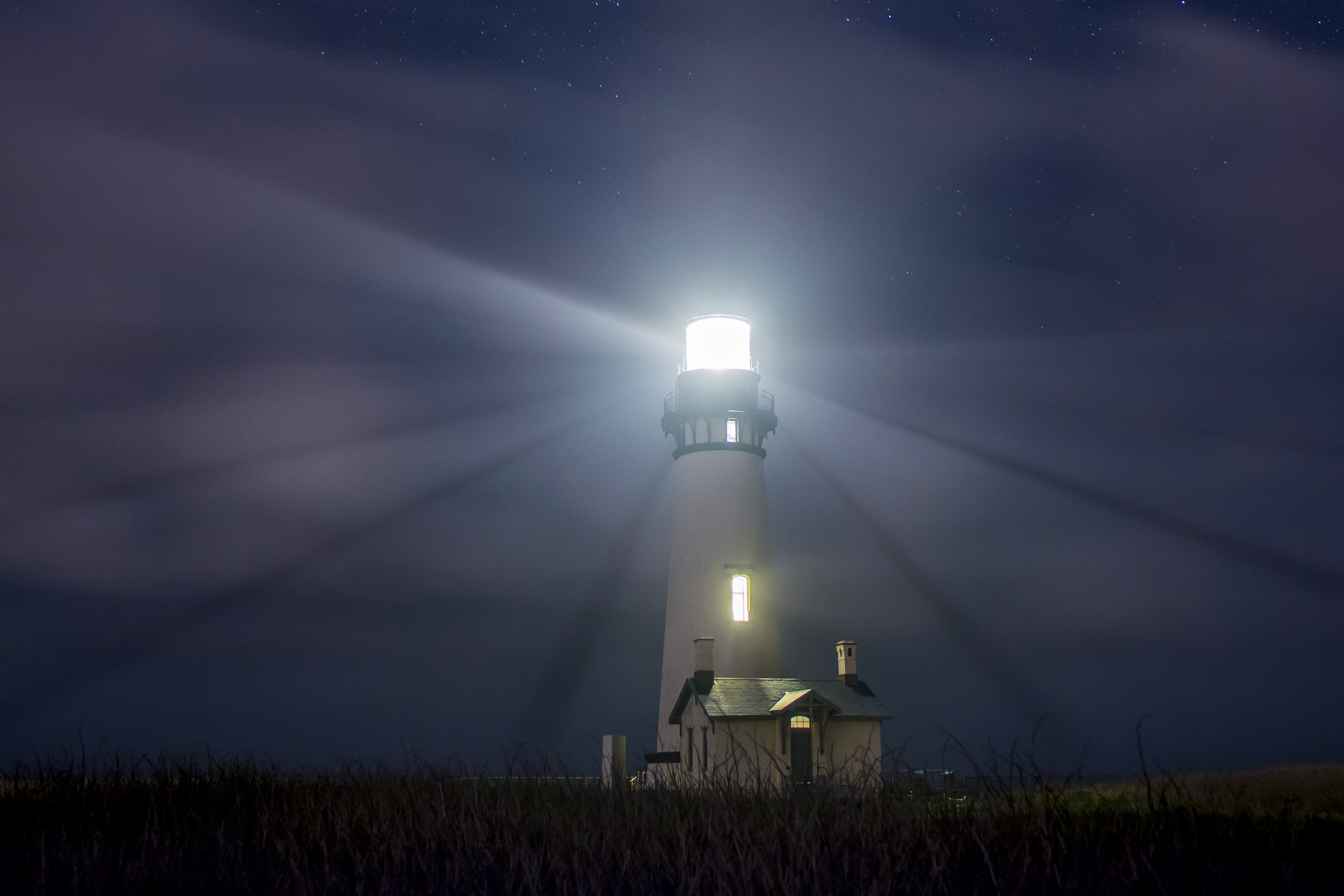
Linear Regression Models
- Linear Regression (LR) is the first and basic statistical tool an economics student comes across in Econometrics. It establishes a straightforward relationship between the independent and dependent variables. Dependent variable and Independent variable notation keep on changing in different circumstances; (Y or X). For example, Y can be consumption...
Limited Dependent Variable Models
- Sometimes we come across dependent variables with discrete and finite or continuous variables with several responses having a threshold limit. For example, the discrete choice of whether someone has a car or not can have two responses. Yes or no. How many hours someone worked on a day can have a continuous range of values from 0-24 hours. Limited dependent variable mo…
Count Data Models
- Count data models have a dependent variable that is counts (0, 1, 2, 3, and so on). Most of the data are concentrated on a few small discrete values. Examples include the number of children a couple has, the number of dentist visits per year a person makes, and the number of education trips per month that a school undertakes. Some of the models are; 1. Poisson model 2. Negativ…
Survival Analysis
- Survival analysis, time-to-event analysis is applied when the data set includes subjects that are tracked until an event happens (failure) or we lose them from the sample. We are interested in how long the observation stays in the sample. Examples include how long a billionaire stays on the Forbes billionaire list, loan performance, and default, death of a patient under treatment. Ass…
Principal Component Analysis
- Principal Component Analysis employs data reduction methods to re-express multivariate data with fewer dimensions. This method is used after conducting surveys to “uncover” the common factors or obtain fewer components to use in subsequent analysis. The reduced data set includes features of original data, causing the highest variance. The feature that causes the second-highe…
Instrumental Variables Model
- Instrumental variable procedures are needed when some regressors are endogenous (correlated with the error term). The procedure for correcting this endogeneity problem involves finding instruments that are correlated with the endogenous regressors but uncorrelated with the error term. Then the two-stage least squares procedure can be applied. An example of instrumental v…
Seemingly Unrelated Regressions Models
- Seemingly unrelated regressions models invented by Gellner in 1962, uses multiple equations instead of a single equation which can be regressed and the estimator can be found independently. it is called on related seemingly because these are only related to error terms. Example: Suppose a country has 10 states and the objective is to study the saving pattern of th…
Time Series Arima Models
- Time series ARIMA (Auto-Regressive Integrated Moving Average) models are applied with time-series data of variables measured over time. It has the following features: A regressive model is a model which uses lacked dependent variable as an independent variable Moving average model: where the mean regression back to its original one integrated when it ages nonstationary to the …