
The expression "treatment effect" refers to the causal effect of a given treatment or intervention (for example, the administering of a drug) on an outcome variable of interest (for example, the health of the patient).
What is a 'treatment effect?
A ‘treatment effect’ is the average causal effect of a binary (0–1) variable on an outcome variable of scientific or policy interest. The term ‘treatment effect’ originates in a medical literature concerned with the causal effects of binary, yes-or-no ‘treatments’, such as an experimental drug or a new surgical procedure.
What is the average treatment effect in research?
Abstract. In randomized clinical trails (RCTs), effect sizes seen in earlier studies guide both the choice of the effect size that sets the appropriate threshold of clinical significance and the rationale to believe that the true effect size is above that threshold worth pursuing in an RCT. That threshold is used to determine the necessary ...
What is the average treatment effect (ATE)?
What is the conditional average treatment effect?

What is meant by treatment effect?
The expression "treatment effect" refers to the causal effect of a given treatment or intervention (for example, the administering of a drug) on an outcome variable of interest (for example, the health of the patient).
What is treatment on the treated effect?
the treatment effect on the treated group equals the treatment effect on the control group (layman terms: people in the control group would do as good as the treatment group if they were treated).Oct 25, 2017
How do you find the treatment effect?
When a trial uses a continuous measure, such as blood pressure, the treatment effect is often calculated by measuring the difference in mean improvement in blood pressure between groups. In these cases (if the data are normally distributed), a t-test is commonly used.
How large is treatment effect?
An effect size is a statistical calculation that can be used to compare the efficacy of different agents by quantifying the size of the difference between treatments. It is a dimensionless measure of the difference in outcomes under two different treatment interventions.
What is treatment effect in Anova?
The ANOVA Model. A treatment effect is the difference between the overall, grand mean, and the mean of a cell (treatment level). Error is the difference between a score and a cell (treatment level) mean.
What is estimate of treatment effect?
Estimates of treatment effect size can be adjusted on the basis of baseline risk to determine the probability that treatment will help a particular patient. The probability that the treatment will be helpful should be weighed against the costs and risks of the treatment.
How precise is the treatment effect?
Recalling that the observed treatment effect is only an estimate of the true effect of the intervention, we would like to have some measure of the uncertainty surrounding the treatment estimate. This precision is usually communicated with a 95% confidence interval (CI).
What is treatment effect in epidemiology?
The estimated treatment effect is the odds ratio comparing the condition that all patients were treated by the therapy of interest with the condition that none of the population was thus treated after adjustment for known covariates.
How do you read hedge G?
As a rule of thumb, here is how to interpret Hedge's g:0.2 = Small effect size.0.5 = Medium effect size.0.8 = Large effect size.Mar 17, 2021
How to estimate conditional average treatment effects?
One way an analyst can estimate conditional average treatment effects is subclassification, which splits observed individuals into subclassifications along variation in their respective values of confounding variables. For example, if an analyst wishes to understand the effect of their treatments on different genders, they may split their population of observed individuals by gender, and estimate causal effects within each defined gender. Once observed individuals have been split into subclassifications, an analyst can estimate causal effects within these groups by leveraging the simple difference in mean outcomes (SDO) calculation discussed in my post covering average treatment effect estimation. As the name suggests, leveraging the SDO requires an analyst to calculate the difference in the mean outcomes within each group with value#N#≈ z#N#color {#7A28CB} approx z ≈ z in order to estimate#N#τ ( z)#N#color {#7A28CB} tau (z) τ (z). An analyst can use this to estimate CATE as a result of a useful theorem from probability theory#N#E [ A − B] = E [ A] − E [ B]#N#E [A - B] = E [A] - E [B] E[A−B]= E[A]−E[B], and thus:
What is heterogeneous treatment effect estimation?
One of the most fruitful applications of heterogeneous treatment effect estimation is within the analytics of automated bidding systems for digital advertisements. Many businesses using digital advertising to expand their customer base use automated bidding systems to purchase ads programmatically on a digital advertisement platform, such as Google Ads. These platforms provide markets with an option to automatically bid for the opportunity to show an ad to a particular user, given a provided set of their characteristics. This purchasing model is quite different then traditional advertising marketplaces, such as those used for television, billboard, and newspaper ads. In those marketplaces, a marketer pays a lump sum to purchase an advertising opportunity accessing a particular swath of the population, (ESPN fans, Highway 101 commuters, New York Times readers) and is only really concerned with how many consumers purchase their product once they are exposed to the purchased ad. On the other hand, when a marketer designs an automated bidding system to interact with digital advertising platforms, they can purchase advertising opportunities which target specific individuals most likely to subsequently convert (which means to visit the marketer’s site and take a revenue-generating action). In order to maximize the resultant profits of an advertising campaign, marketers often try to estimate the heterogeneous treatment effects of their campaigns to ensure they only purchase expensive digital advertisements that target consumers most likely to be influenced.
What is the average treatment effect?
The average treatment effect ( ATE) is a measure used to compare treatments (or interventions) in randomized experiments, evaluation of policy interventions, and medical trials. The ATE measures the difference in mean (average) outcomes between units assigned to the treatment and units assigned to the control.
What is treatment in science?
Originating from early statistical analysis in the fields of agriculture and medicine, the term "treatment" is now applied, more generally, to other fields of natural and social science, especially psychology, political science, and economics such as, for example, the evaluation of the impact of public policies.
What is heterogeneous treatment?
Some researchers call a treatment effect "heterogenous" if it affects different individuals differently (heterogeneously). For example, perhaps the above treatment of a job search monitoring policy affected men and women differently, or people who live in different states differently.
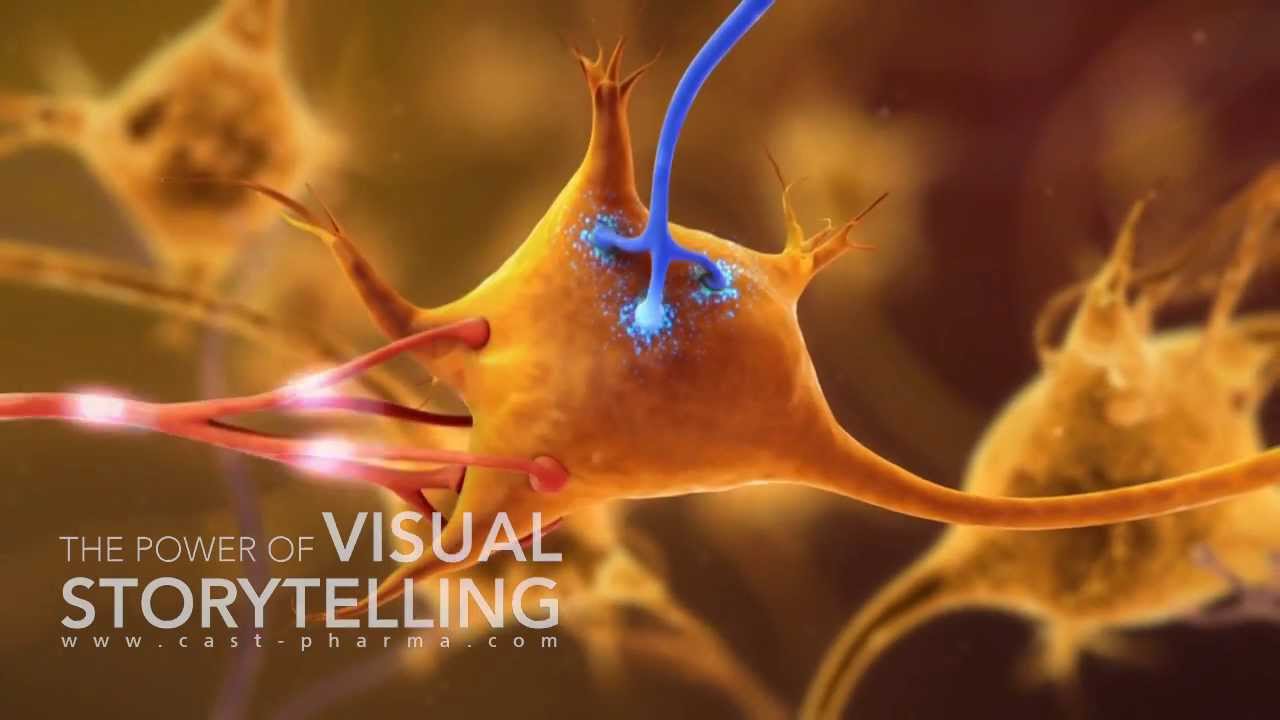
Optimized Bidding For Digital Advertisements
Conditional Average Treatment Effects
- The particular heterogeneous treatment effect I am interested in estimating are conditional average treatment effects (CATE), or the expected treatment effect of a particular consumer conditional on a set of explanatory variables describing them, such as Past Behavior, Demographic Data, and Psychographic Data. Formally, one can define a conditional average trea…
Estimating Conditional Average Treatment Effects
- In order to estimate Yi1−Yi0\color{#EF3E36}Y^1_i - Y^0_iYi1−Yi0 for an individual consumer an analyst will often leverage variation in the value of an explanatory variable Xi\color{#7A28CB}X_iXi of interest as is common with many causal inference methods. Particularly, an analyst will leverage variation in treatment within groups of observed individuals with similar values of conf…
Bias in Estimation of Cate
- Much like any estimation of a causal effect, estimations of conditional average treatment effects are susceptible to distortions from confounding bias, which distorts the estimate of a causal effect by adding additional correlation between an explanatory variable and an outcome variable within a causal relationship of interest. Additionally recall from my confounding bias post that i…
Lifting The Curse
- What machine learning strategies exist for selecting the optimal set of confounding variables for an analyst to condition their CATE estimates on? What is the evaluation metric for such strategies? For example, how would such a strategy identify which confounding variables have the greatest effect on Exposed To Ad and Purchases Flight? All this and more will be revealed in my …
Overview
The average treatment effect (ATE) is a measure used to compare treatments (or interventions) in randomized experiments, evaluation of policy interventions, and medical trials. The ATE measures the difference in mean (average) outcomes between units assigned to the treatment and units assigned to the control. In a randomized trial (i.e., an experimental study), the average treatment effect can be estimatedfrom a sample using a comparison in mean outcomes for treated and un…
General definition
Originating from early statistical analysis in the fields of agriculture and medicine, the term "treatment" is now applied, more generally, to other fields of natural and social science, especially psychology, political science, and economicssuch as, for example, the evaluation of the impact of public policies. The nature of a treatment or outcome is relatively unimportant in the estimation of the ATE—that is to say, calculation of the ATE requires that a treatment be applied to some unit…
Formal definition
In order to define formally the ATE, we define two potential outcomes : is the value of the outcome variable for individual if they are not treated, is the value of the outcome variable for individual if they are treated. For example, is the health status of the individual if they are not administered the drug under study and is the health status if they are administered the drug.
The treatment effect for individual is given by . In the general case, there is no reason to expect th…
Estimation
Depending on the data and its underlying circumstances, many methods can be used to estimate the ATE. The most common ones are:
• Natural experiments
• Difference in differences
• Regression discontinuity designs
An example
Consider an example where all units are unemployed individuals, and some experience a policy intervention (the treatment group), while others do not (the control group). The causal effect of interest is the impact a job search monitoring policy (the treatment) has on the length of an unemployment spell: On average, how much shorter would one's unemployment be if they experienced the intervention? The ATE, in this case, is the difference in expected values (means…
Heterogenous treatment effects
Some researchers call a treatment effect "heterogenous" if it affects different individuals differently (heterogeneously). For example, perhaps the above treatment of a job search monitoring policy affected men and women differently, or people who live in different states differently. ATE requires a strong assumption known as the stable unit treatment value assumption (SUTVA) which requires the value of the potential outcome be unaffected by the me…
Further reading
• Wooldridge, Jeffrey M. (2013). "Policy Analysis with Pooled Cross Sections". Introductory Econometrics: A Modern Approach. Mason, OH: Thomson South-Western. pp. 438–443. ISBN 978-1-111-53104-1.