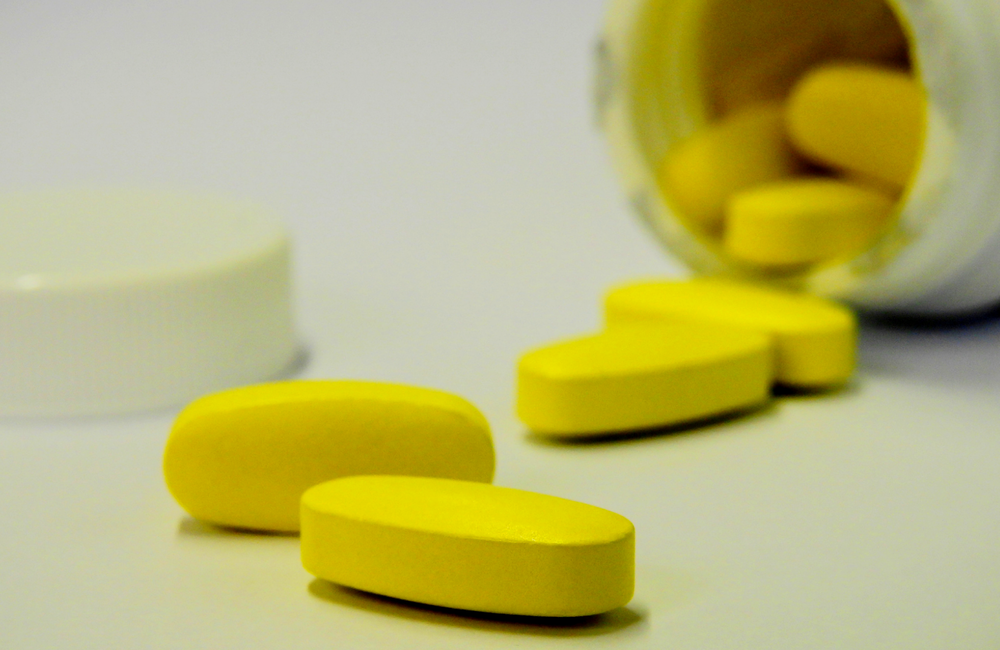
Difference in differences requires data measured from a treatment group and a control group at two or more different time periods, specifically at least one time period before "treatment" and at least one time period after "treatment."
How to calculate the difference-in-diff between treatment post and control post?
as in the following formula: (1.1) (Treatment_post - Treatment_pre) - (Control_post - Control_pre) = Diff-in-Diff estimate We can calculate the difference-in-difference based on graph 1.1, as below:
How does the coefficient β2 (post-treatment) differ from zero?
The coefficient β 2 (Post-treatment) is significant and different from zero. We can conclude that the price of houses in the control group increased from time = 1 to time = 2. The average price of houses in the control group increases of 35,230. 86$ at time = 2. The average price of houses in the control group at time = 2 is 216,672. 6 + 35,230.
How do you calculate the difference between treatment effect and trend?
the difference in the treatment group before and after the treatment (the treatment effect) the difference in the control group before and after the treatment (the trend over time) as in the following formula: (1.1) (Treatment_post - Treatment_pre) - (Control_post - Control_pre) = Diff-in-Diff estimate
How common are post-treatment trends in did estimates?
This is likely to be common; when you see “event study” DiD estimates, the post-treatment period usually shows post-treatment trends in the estimated effects.
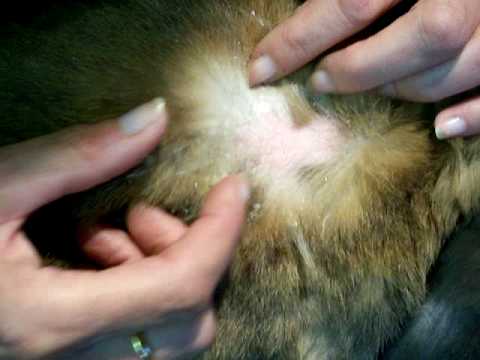
How do you explain difference in differences?
The difference-in-differences method is a quasi-experimental approach that compares the changes in outcomes over time between a population enrolled in a program (the treatment group) and a population that is not (the comparison group). It is a useful tool for data analysis.
What is the counterfactual in difference in difference?
Counterfactual Assumption (2b) essentially disregards time points other than these two. That is, the other time points need not satisfy any “parallel trends” assumption. While this assumption is perfectly valid if true, using such an assumption requires justification.
What is a difference in difference regression?
Difference in differences (DiD) is a non-experimental statistical technique used to estimate treatment effects by comparing the change (difference) in the differences in observed outcomes between treatment and control groups, across pre-treatment and post-treatment periods.
What is a difference in difference estimation?
Difference-in-differences estimation attempts to measure the effects of a sudden change in economic environment, policy, or general treatment on a group of individuals. The DD model includes several pieces: A sudden exogenous source of variation, which we will refer to as the treatment.
What is generalized diff in diff?
The modified DD is a generalized difference in differences (GDD), which is a DD with one additional time-wise difference. GDD allows the selection effect to be a constant that is not necessarily zero, and the constant is removed by the additional time-wise difference using the two pretreatment periods.
What is staggered difference in difference?
Difference-in-differences analysis with staggered treatment timing is frequently used to assess the impact of policy changes on corporate outcomes in academic research. However, recent advances in econometric theory show that such designs are likely to be biased in the presence of treatment effect heterogeneity.
Why is difference in differences used?
Hence, Difference-in-difference is a useful technique to use when randomization on the individual level is not possible. DID requires data from pre-/post-intervention, such as cohort or panel data (individual level data over time) or repeated cross-sectional data (individual or group level).
What is a difference in differences in research?
Difference in differences (DID or DD) is a statistical technique used in econometrics and quantitative research in the social sciences that attempts to mimic an experimental research design using observational study data, by studying the differential effect of a treatment on a 'treatment group' versus a 'control group' ...
What is the difference between OLS and difference?
Difference in difference refers to an empirical strategy or model where some treatment effect is estimated by comparing changes in the treatment group over time to changes in the control group over time. The model is typically a linear regression model estimated using ordinary least squares.
What is analysis of difference?
1. For example, difference analysis is used to see whether there are differences between or among groups of people or types of texts. 2. In each case, the independent variable is measured using a nominal scale and the. research question or hypothesis is about the differences between the nominal categories.
What is a first difference model?
The first-differenced (FD) estimator is an approach that is used to address the problem of omitted variables in econometrics and statistics by using panel data. The estimator is obtained by running a pooled OLS estimation for a regression of the differenced variables.
Did with variation in treatment timing?
The canonical difference-in-differences (DD) model contains two time periods, “pre” and “post”, and two groups, “treatment” and “control”. Most DD applications, however, exploit variation across groups of units that receive treatment at different times.
What are the implications of exogeneity?
First, strict exogeneity requires that the treatment, T, and the covariates, X, be uncorrelated with the time-varying errors in every time period. This precludes situations where, say, current political institutions respond to past (unobserved) idiosyncratic shocks (so-called Ashenfelter's dip). Strict exogeneity is a strong assumption. Second, the rank condition rules out observable covariates that do not vary over time; it also requires that the treatment itself varies over time (which may be problematic for questions involving many political institutions). If one assumes that such time invariant covariates have identical effects on both potential outcomes (i.e., β1 = β0 for these observables), then this is not problematic for estimating the parameters in eqn [6]; these covariates simply drop out of eqn [20]. However, absent this assumption, the parameters of interest are no longer identified. Finally, DD estimators identify the causal effect of the treatment from onetime changes in outcomes that occur at the time observations switch from the treatment to the control group (or vice versa). As such, it is imperative that the timing of the treatment be measured correctly and that the treatment does not alter environmental policy before (due to anticipatory effects) or after (due to lagged effects) the measured date of the treatment. In the context of analyzing political institutions, this point is particularly salient since institutional changes are not likely to be unexpected.
What is the DID methodology?
The DID methodology is often used in the banking literature and elsewhere to compare a treatment group to a control group before and after treatment. For the TARP research, the treatment group usually consists of banks that received TARP funds, and the control group consists of other banks that did not receive the funds. 1 In some of the research, the treatment is at the state level—the proportion of banks in the state that received TARP bailouts. In some cases, treatment is at the individual loan level, comparing the terms of credit on loans from TARP banks with those from non-TARP banks before and after the TARP treatment. For expositional purposes, we begin with the DID model at the bank level, which typically takes the form:
What happens in the first period of a study?
In the first period, none of the groups is exposed to treatment. In the second period, only one of the groups gets exposed to treatment, but not the other. To provide an illustration, suppose that there are two classes in a given school observed at the beginning and the end of a school year.
What is the DD method?
The DD method has been used in hundreds of studies in economics, especially in the last two decades, but the basic idea has a long history. An early example in labor economics is Lester (1946), who used the differences-in-differences technique to study employment effects of minimum wages. 14.
What is the impact of a treatment?
Basically, the impact of a treatment is the difference in outcomes between the treatment and control groups, after the project is implemented, taking into account all the already-existing differences in outcomes between the treatment and control groups. It is usual to define a treatment as a form of policy intervention.
What is DD in statistics?
Difference-in-differences (DD) methods attempt to control for unobserved variables that bias estimates of causal effects, aided by longitudinal data collected from students, school, districts, or states . Researchers employ two varieties of longitudinal data. Panel data track the progress of the same students or teachers in successive months or years. Repeated cross-section data follow different groups of individuals (e.g., second-graders in successive years) that are clustered within the same schools, districts, or states.
How to determine whether a particular intervention has an impact on our target population or on a specific target outcome?
To examine whether a particular intervention has an impact on our target population or on a specific target outcome, we use an econometric approach known as the difference-in-difference procedure. The difference-in-difference analysis helps us to answer the counterfactual question: what would have happened to the outcome, if the said intervention had not taken place? If the counterfactual question can be answered, then one can compare this answer to the factual situation, where the intervention or the treatment was initiated. The true impact of the treatment would then be the difference between the factual values and the answer to the counterfactual question.
When was the difference in difference technique invented?
The difference-in-difference (DID) technique originated in the field of econometrics, but the logic underlying the technique has been used as early as the 1850’s by John Snow and is called the ‘controlled before-and-after study’ in some social sciences.
What is a DID?
DID is a quasi-experimental design that makes use of longitudinal data from treatment and control groups to obtain an appropriate counterfactual to estimate a causal effect. DID is typically used to estimate the effect of a specific intervention or treatment (such as a passage of law, enactment of policy, or large-scale program implementation) ...
What is the purpose of the phrase "did"?
DID usually is used to estimate the treatment effect on the treated (causal effect in the exposed), although with stronger assumptions the technique can be used to estimate the Average Treatment Effect (ATE) or the causal effect in the population. Please refer to Lechner 2011 article for more details.
What is the purpose of the DID?
DID is used in observational settings where exchangeability cannot be assumed between the treatment and control groups. DID relies on a less strict exchangeability assumption, i.e., in absence of treatment, the unobserved differences between treatment and control groups arethe same overtime.
1 The key concept
The difference-in-difference (diff-in-diff) is a powerful model which allows us to look at the effect of a policy intervention by taking into consideration:
2 A Difference-in-Difference model
The U.S. Dept. of Housing and Urban Development, along with state and local governments, subsidize several housing projects to create affordable living spaces. This policy has become increasingly important in the past yeas, as rent and housing costs increase in several US cities.
3 Replication of a study
We are going to replicate a study conducted by Card and Krueger in 1994 that investigate the relationship between a rise in minimum wage and employment.
4 Additional resources
In this section we are going to look at some more cases of difference-in-difference as well as a key assumption that you need to consider.
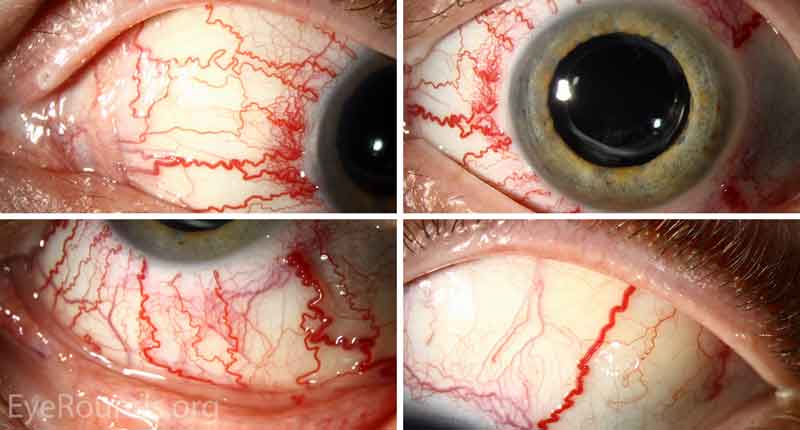