
Nonrandomized pharmacoepidemiology generally compares one medication with another. For many conditions, clinicians can benefit from comparing the safety and effectiveness of three or more appropriate treatment options. We sought to compare three treatment groups simultaneously by creating 1:1:1 propensity score-matched cohorts.
Full Answer
Can propensity score methods be extended to multiple treatment cases?
For many conditions, clinicians can benefit from comparing the safety and effectiveness of three or more appropriate treatment options. We sought to compare three treatment groups simultaneously by creating 1:1:1 propensity score-matched cohorts. Methods: We developed a technique that estimates generalized propensity scores and then creates 1:1:1 matched sets. …
How many groups are needed for propensity score weighting?
Asked 27th May, 2016. Gabriel Lawin. I have 3 treatments, saying A, B and C. I estimated the propensity score for each treatment using mlogit model and …
What is propensity score and how to use it?
Mar 29, 2012 · The algorithm requires two generalized propensity scores for each patient: probabilities of being treated with each of Treatments 1 and 2, which together determine the probability of Treatment 3. We estimated these propensity scores using multinomial logistic regression. 8, 17 The algorithm we created matches without replacement and performs “within …
Do propensity score weights improve balance on observed pretreatment covariates?
Jan 29, 2015 · It would be ideal to have a well-written, easily-accessed on-line tutorial that illustrates the running of a three-group propensity model from beginning to end. Also, the three groups in my data set are of badly unbalanced size -- the respective numbers of cases in the three groups are about 250, 150, and 46.

How many variables should be in propensity score?
How is propensity score calculated?
What is propensity score matching cohort study?
What is the minimum sample size for propensity score matching?
When is a propensity score used?
What is propensity value?
What is propensity Modelling?
What is propensity score in statistics?
How do you match propensity scores in Excel?
What is propensity score weighting?
Does matching increase sample size?
Abstract
Nonrandomized pharmacoepidemiology generally compares one medication with another. For many conditions, clinicians can benefit from comparing the safety and effectiveness of three or more appropriate treatment options. We sought to compare three treatment groups simultaneously by creating 1:1:1 propensity score-matched cohorts.
METHODS
A three-group study of Treatments 1, 2, and 3 yields three possible effects of interest: 1 versus 2, 2 versus 3, and 1 versus 3. We considered three approaches to estimation of the treatment effects. We began with a simple pairwise approach in which we assessed the three possible contrasts separately.
RESULTS
We ran 1000 simulations per combination of input parameters. Across all simulation runs ( Table 1 ), including those with strong unmeasured confounding, we observed a bias in the unmatched cohort ranging from 247% to 764%, and an MSE (×100) of 6.16–58.46.
DISCUSSION
We developed and tested an algorithm for simultaneously matching groups of three patients on propensity score, to create cohorts of exchangeable patients among whom the comparative effects of three exposure groups could be studied.
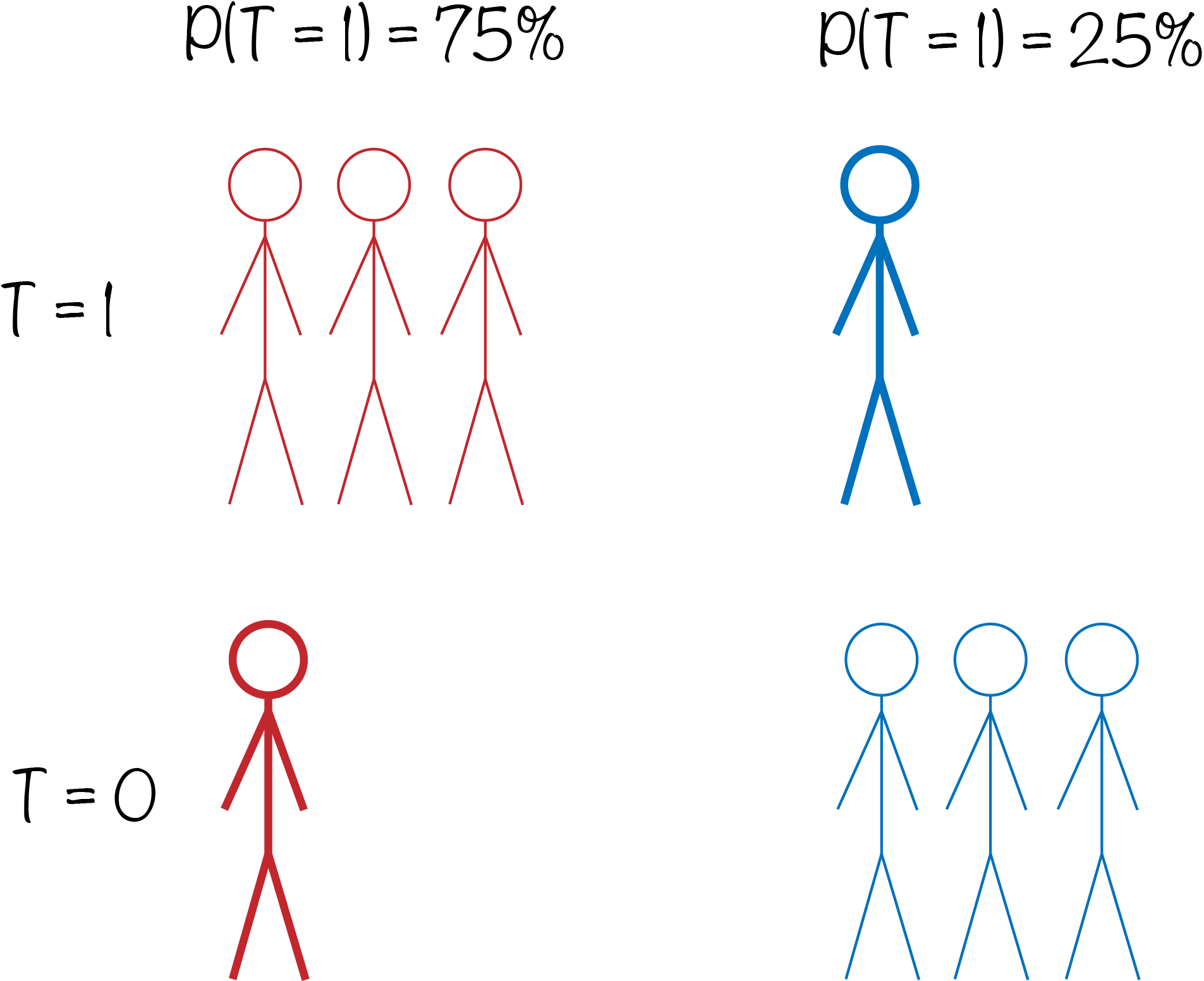