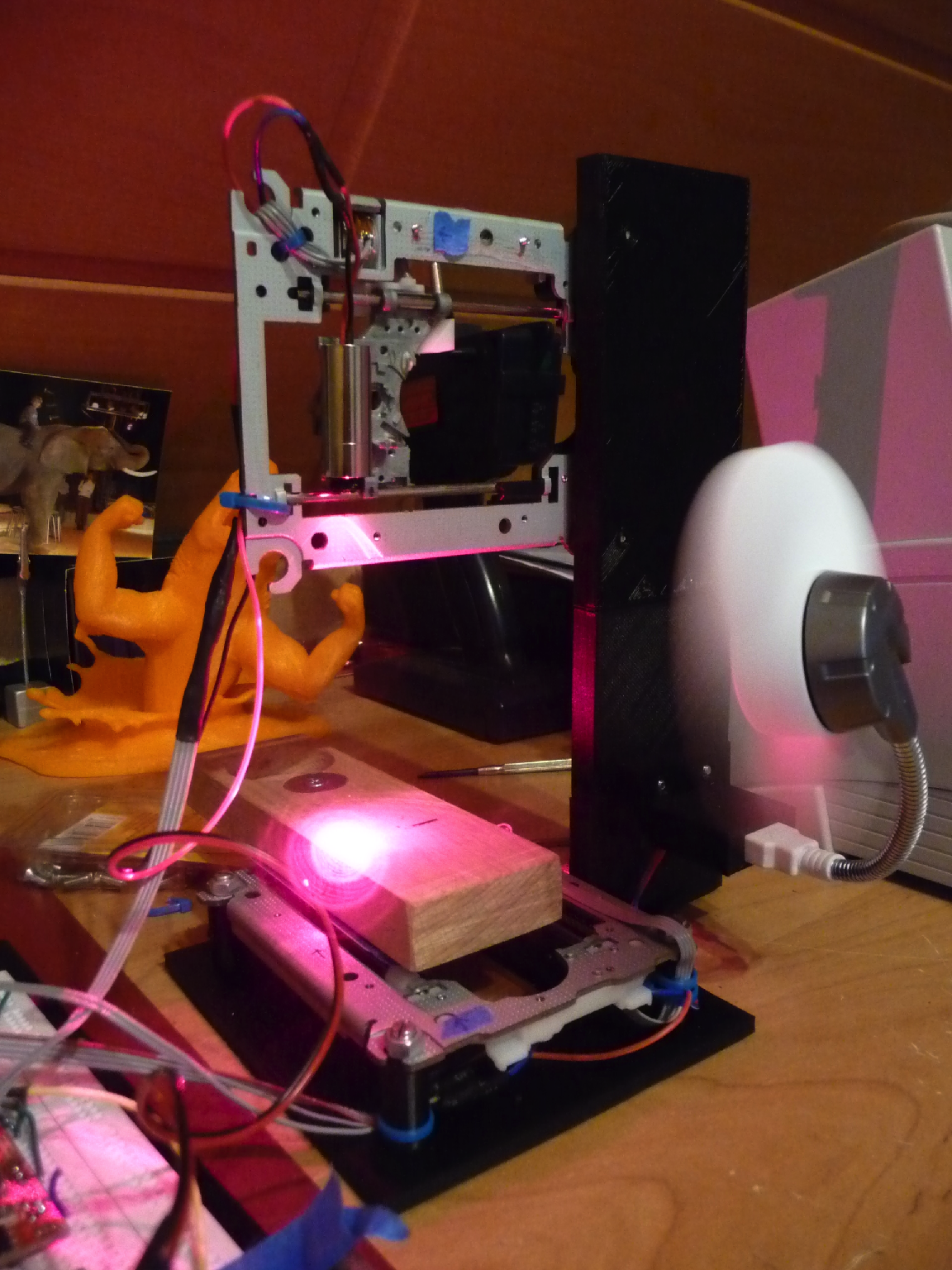
What is the difference between wavelength bands in multispectral imaging?
· Feature selection is an essential step in hyperspectral image classification due to the small number of labeled samples versus the high number of features which cause the Hughes phenomenon. Feature selection consists of the elimination of irrelevant and highly correlated features. In this paper, we present a framework for the feature selection ...
What is hyperspectral imaging and how does it work?
Band selection methods are designed to select a subset of hyperspectral spectral features to remove spectral redundancy, while retaining the important information of the entire image [7].
What are the four primary hyperspectral acquisition modes?
· Taking the quality (Q) of the data cube as selection criterion, MNBS tries to find the bands with both high SNRs and low correlation (high ). The subset selection method, …
What is the HPLC/ESI-MS/MS screening method?
The proposed band selection methodology is. also applicable to other application domains requiring hyperspectral data analysis. In the future, we would like to improve the unsupervised …
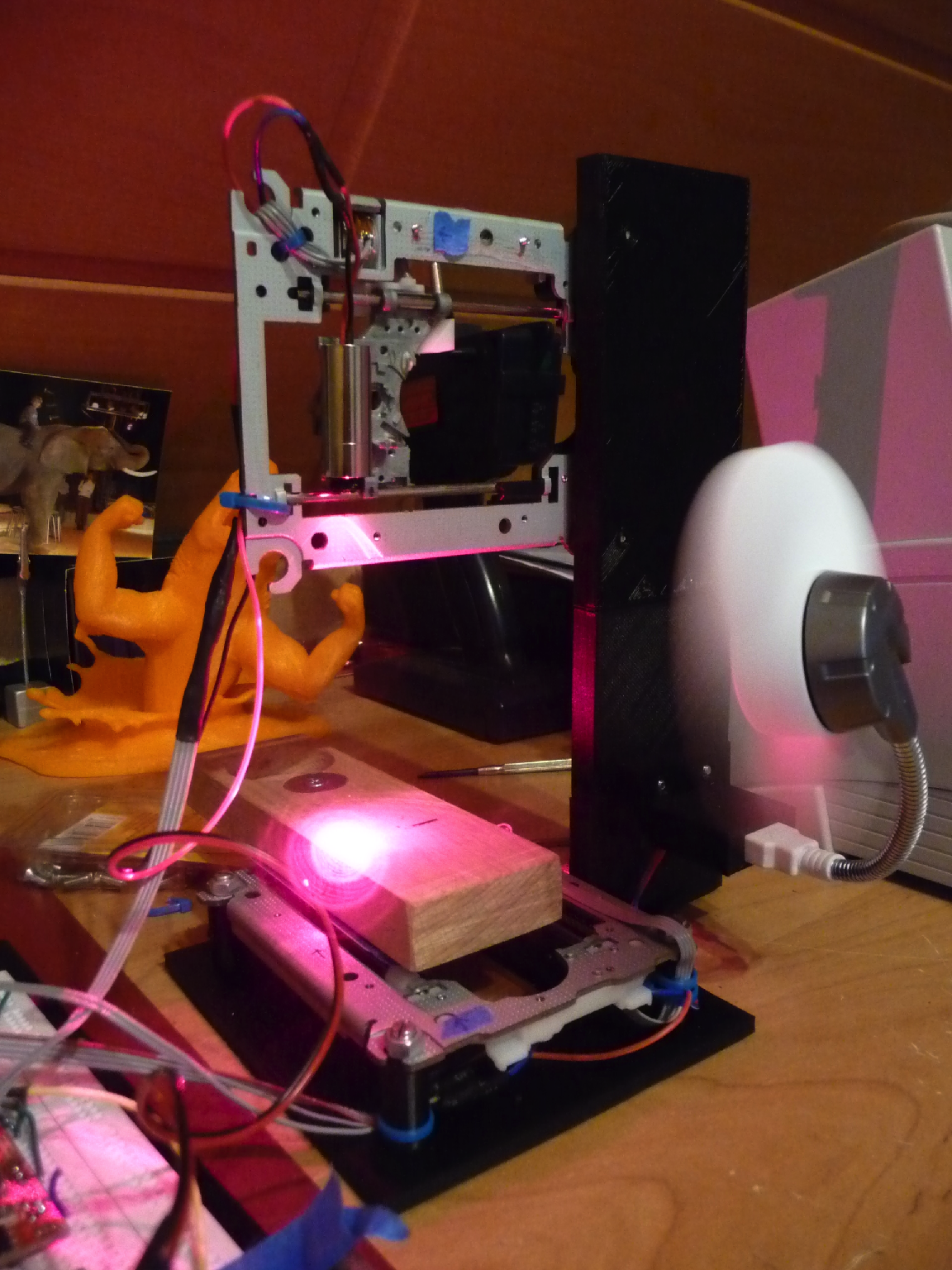
Abstract
Feature selection is an essential step in hyperspectral image classification due to the small number of labeled samples versus the high number of features which cause the Hughes phenomenon. Feature selection consists of the elimination of irrelevant and highly correlated features.
1. Introduction
Recently, hyperspectral image classification has become a very interesting field of research in remote sensing and other applications ( Ji et al., 2014, Yang et al., 2014a, Yang et al., 2014b, Medjahed et al., 2016 ). It aims to classify each pixel in a hyperspectral image into a specific category.
2. The proposed band selection method
The aim of hyperspectral image classification is to classify each pixel of a spectral image into a specific class. We denote the classes set Y = {C1, C2, …, Cm} and X = {X1, X2, …, Xn} as the features/bands set. We define the set F = {f1, f2, …, fn}, which determines the selected features:
4. Conclusions
In this paper, we have described a new framework for feature selection in the context of hyperspectral image classification. The problem of feature selection is cast as a minimization procedure.
What is the challenge of hyperspectral imaging?
An important challenge in hyperspectral imaging tasks is to cope with the large number of spectral bins. Common spectral data reduction methods do not take prior knowledge about the task into account. Consequently, sparsely occurring features that may be essential for the imaging task may not be preserved in the data reduction step. Convolutional neural network (CNN) approaches are capable of learning the specific features relevant to the particular imaging task, but applying them directly to the spectral input data is constrained by the computational efficiency. We propose a novel supervised deep learning approach for combining data reduction and image analysis in an end-to-end architecture. In our approach, the neural network component that performs the reduction is trained such that image features most relevant for the task are preserved in the reduction step. Results for two convolutional neural network architectures and two types of generated datasets show that the proposed Data Reduction CNN (DRCNN) approach can produce more accurate results than existing popular data reduction methods, and can be used in a wide range of problem settings. The integration of knowledge about the task allows for more image compression and higher accuracies compared to standard data reduction methods.
How does band selection work?
Band selection refers to the process of choosing the most relevant bands in a hyperspectral image. By selecting a limited number of optimal bands, we aim at speeding up model training, improving accuracy, or both. It reduces redundancy among spectral bands while trying to preserve the original information of the image. By now, many efforts have been made to develop unsupervised band selection approaches, of which the majorities are heuristic algorithms devised by trial and error. In this article, we are interested in training an intelligent agent that, given a hyperspectral image, is capable of automatically learning policy to select an optimal band subset without any hand-engineered reasoning. To this end, we frame the problem of unsupervised band selection as a Markov decision process, propose an effective method to parameterize it, and finally solve the problem by deep reinforcement learning. Once the agent is trained, it learns a band-selection policy that guides the agent to sequentially select bands by fully exploiting the hyperspectral image and previously picked bands. Furthermore, we propose two different reward schemes for the environment simulation of deep reinforcement learning and compare them in experiments. This, to the best of our knowledge, is the first study that explores a deep reinforcement learning model for hyperspectral image analysis, thus opening a new door for future research and showcasing the great potential of deep reinforcement learning in remote sensing applications. Extensive experiments are carried out on four hyperspectral data sets, and experimental results demonstrate the effectiveness of the proposed method. The code is publicly available.
What is band selection?
Band selection and feature extraction are the two paradigms of hyperspectral dimensionality reduction. While feature extraction methods have many desirable properties, band selection methods keep the actual reflectances intact which leads to better interpretation of the physical properties of the materials. Keeping this in mind, several feature extraction-based band selection methods have been proposed in the past which can bridge the gap between these two approaches and combine their salient advantages. In this paper, a feature-extraction based clustering-ranking type band selection method is proposed in which band selection is performed in two stages. In the Clustering stage, new and improved representation of bands using their component loadings for the top principal components are used instead of their original reflectances to cluster similar bands into groups using Sparse Subspace Clustering which has resulted in effective clustering results. In the Ranking stage, a new metric called Combined Divergence-Correlation Index (CDCI) is introduced to elect cluster representative bands which are jointly the most discriminative as well as least correlated among each other. Experimental results indicate that the proposed method can adeptly select a set of distinct and discriminative bands which can help in effective hyperspectral classification. Additionally, it was also shown experimentally that the selected bands show rich information content, low inter-band correlations and high class separabilities.
How does a hyperspectral sensor work?
A hyperspectral imaging sensor collects detailed spectral responses from ground objects using hundreds of narrow bands; this technology is used in many real-world applications. Band selection aims to select a small subset of hyperspectral bands to remove spectral redundancy and reduce computational costs while preserving the significant spectral information of ground objects. In this article, we review current hyperspectral band selection methods, which can be classified into six main categories: ranking based, searching based, clustering based, sparsity based, embedding-learning based, and hybrid-scheme based. With two widely used hyperspectral data sets, we illustrate the classification performances of several popular band selection methods. The challenges and research directions of hyperspectral band selection are also discussed.
What is BS in spectral image?
Hyperspectral image (HSI) band selection (BS) is an important task for HSI dimensionality reduction, whose goal is to select an informative band subset containing less redundancy. However, traditional BS methods basically work in the Euclidean domain and thus often neglect to consider the structural information of spectral bands. In this paper, to make full use of the structural information, a novel BS method termed as Efficient Graph Convolutional Self-Representation (EGCSR) is proposed by incorporating graph convolution into the self-representation model. Since the proposed method is typically modeled in the non-Euclidean domain, it tends to result in a more robust self-representation coefficient matrix. We provide a closed-form solution to the EGCSR model, which leads to high computational efficiency. We further propose two strategies to determine the informative band subset from the coefficient matrix. The first is a ranking-based strategy (EGCSR-R), which ranks every band by calculating the cumulative contribution, and the second is a clustering-based strategy (EGCSR-C), which treats band selection as a band clustering task based on using subspace segmentation. Extensive experimental results on three real HSI data sets show that the proposed EGCSR model is dramatically superior to many existing band selection methods and with high computational efficiency.
How does semi-supervised learning work?
Semi-supervised learning is an effective technique to represent the intrinsic features of hyperspectral image (HSI), which can reduce the cost to obtain the labeled information of samples. However, traditional semi-supervised learning methods fail to consider multiple properties of HSI, which have restricted the discriminant performance of feature representation. In this paper, we introduce the hypergraph into semi-supervised leraning to reveal the complex multi-structures of HSI, and construct a semi-supervised discriminant hypergraph learning (SSDHL) method by designing an intraclass hypergraph and an interclass graph with the labeled samples. SSDHL constructs an unsupervised hypergraph with the unlabeled samples. In addition, a total scatter matrix is used to measure the distribution of the labeled and unlabeled samples. Then, a low-dimensional projection function is constructed to compact the properties of the intraclass hypergraph and the unsupervised hypergraph, and simultaneously separate the characteristics of the interclass graph and the total scatter matrix. Finally, according to the objective function, we can obtain the projection matrix and the low-dimensional features. Experiments on three HSI data sets (Botswana, KSC, and PaviaU) show that the proposed method can achieve better classification results compared with a few state-of-the-art methods. The results indicate that SSDHL can simultaneously utilize the labeled and unlabeled samples to represent the homogeneous properties and restrain the heterogeneous characteristics of HSI.
How does mass spectrometry work?
Mass spectrometry is an analytical technique that can provide both qualitative (structure) and quantitative (molecular mass or concentration) information on analyte molecules after their conversion to ions. The molecules of interest are first introduced into the ionisation source of the mass spectrometer, where they are first ionised to acquire positive or negative charges. The ions then travel through the mass analyser and arrive at different parts of the detector according to their mass/charge (m/z) ratio. After the ions make contact with the detector, useable signals are generated and recorded by a computer system. The computer displays the signals graphically as a mass spectrum showing the relative abundance of the signals according to their m/z ratio.
Which type of monitoring is used for ESI-MS/MS?
Multiple reaction monitoring: Both Q1 and Q3 are static for a pre-determined pair of precursor and product ions. This confers the highest specificity and sensitivity and is commonly used in ESI-MS/MS quantification procedures.
What is ESI-MS?
It provides a sensitive, robust, and reliable tool for studying, at femto-mole quantities in micro-litre sample volumes, non-volatile and thermally labile bio-molecules that are not amenable to analysis by other conventional techniques. Coupled with a high performance liquid chromatograph (HPLC) for molecular fractionation prior to mass spectrometric analysis, HPLC/ESI-MS has become a very powerful technique capable of analysing both small and large molecules of various polarities in a complex biological sample. With the additional separation capabilities of tandem mass spectrometry (MS in a series, commonly denoted as MS/MS), complicated sample purification and procedures for derivative formation commonly used in gas chromatography (GC)-MS can be much simplified. Together with automated sample introduction, HPLC/ESI-MS/MS is a flow analysis technique for rapid analysis and high sample throughput. The focus of MS manufacturers in recent years has been on the development of MS analysers controlled by user-friendly computer software. Clinical biochemists and other biomedical scientists can manage this technique without an in-depth understanding of the complicated physical processes and mathematics principles. This article aims to provide a basic understanding of the ESI process, mass analysers, data acquisition, and specific requirements for qualitative and quantitative molecular analysis. Methods for the study of inborn errors of metabolism (small molecules) and haemoglobin variants (macromolecules) will be summarised for illustration of clinical applications.
What is the mass spectrum?
The mass spectrum is a graphical display of the relative a bundance of ion signals against the m/z ratios. It is a common practice that the highest signal is taken as 100% abundance and all the other signals are expressed as a percentage of this. Fragmentation of the protonated or de-protonated molecular ions generated from ESI is generally limited, and the mass spectra are relatively simple. However, multiple-protonation of proteins and peptides occurs in the ESI process, and the ESI mass spectra might become more complicated. Figure 5is the mass spectrum for a highly purified horse myoglobin preparation (5 μmol/L in 50% acetonitrile containing 0.2% formic acid). A single protein will show its characteristic cluster ions containing multiple-charged ions. The number of charges on the protein molecules will depend on the molecular weight of the protein and the number of accessible basic sites (e.g. arginine, histidine, and lysine). Three basic assumptions are made in the determination of the molecular weight of a single protein. Namely, that (1) adjacent peaks in a series only differ by one charge; (2) charging is only due to proton attachment to the molecular ions; and (3) ionisation occurs to only the intact molecule. To calculate the molecular weight of the protein, one can use 2 simultaneous equations derived from 2 adjacent ions. As an example, for the ion with a m/z ratio = 1212, it has an adjacent peak with m/z ratio = 1131. The adjacent peak must have an additional charge on the molecular ions. Therefore, 2 simultaneous equations can be set up: m/z = 1212, and m/(z+1) = 1131, solving to z = 14 and m = 16968 for the molecule.
What is the mass spectrum of a mixture of serum alpha-foetal proteins purified by affinity
Mass spectrum of a mixture of serum alpha-foetal proteins purified by affinity chromatography. The top spectrum comprises the raw data showing the composite cluster ions of the different glycoforms. The bottom spectrum is the deconvoluted spectrum on the true mass scale after the Micromass MaxEnt processing.
How to separate ions in MS?
When ions travel through a magnetic or electrical field, their movement is affected by their m/z ratio and this is the main principle of separating ions in MS. In the clinical laboratory, a quadrupole mass analyser is most commonly used. In such system, an assembly of 4 parallel metal rods is kept at equal distance (Figure 2). Each pair of opposite rods is connected electrically. An equal but opposite DC voltage superimposed with a radio frequency (RF) AC voltage is applied to the diagonally placed pair of rods. The resulting electrical field causes the ions to travel forward in the z direction with oscillatory motion in the x-y plane. The amplitude of oscillation bears a unique relationship with the m/z ratio and can be controlled by changing the DC and RF voltages simultaneously in a pre-fixed ratio. These DC and RF voltages can be set so that amplitudes of oscillation for desirable m/z ratios are “stable” with the ions travelling along the z-axis without hitting the quadrupole rods, and finally reaching the detector. On the other hand, the oscillatory amplitudes of undesirable ions are large and “unstable”; they hit the metal rods, get neutralised, and fail to reach the detector. Quadrupole mass analysers are robust, economical, physically small, and more readily interfaced with a wide variety of inlet systems when compared with other conventional mass analysers like the magnetic sector.
What is the ion signal in ESI-MS?
In ESI-MS, the ion signal is proportional to analyte concentration and largely independent of flow rate and injection volume used for sample introduction.1The signal is linear from the limit of detection (usually pmol/L) to around 10 μmol/L of analyte concentration. For quantitative measurement, it is important to incorporate an internal standard in the procedure to compensate for losses during sample preparation and variable detection sensitivity of the MS system. The internal standard should have a structure similar to that of the analyte and the ideal practice is to synthesise an internal standard by incorporating stable isotopes on the molecules of interest. For example, for the quantification of free carnitine (m = 162), an internal standard containing 3 deuterium atoms to replace 3 hydrogen atoms was used (m = 165).4When an ideal internal standard is not available, molecules with similar structure can also be used. For example, ascomycin has been used as an internal standard for ESI-MS/MS analysis of the immunosuppressant tacrolimus.5
What is the difference between hyperspectral imaging and multispectral imaging?
Figure 4: Comparison of the image stacks in multispectral imaging, in which there are images taken in several different spectra, and hyperspectral imaging, in which there are images taken in many different spectra.
What is hyperspectral imaging?
Hyperspectral and multispectral imaging are two similar technologies that have been growing in prominence and utility over the past two decades. The terms are often conflated to have the same meaning, but represent two distinct imaging methods, each with their own application spaces. Both technologies have advantages over conventional machine ...
What is the peak spectral sensitivities of a camera?
Imaging lens assemblies and sensors typically have peak spectral sensitivities around 550nm. The quantum efficiency of a camera sensor is the ability to convert photons into an electric signal; this efficiency decreases significantly into the ultraviolet or the near infrared. In the simplest terms, hyperspectral imaging (HSI) ...
What are the applications of HSI and MSI?
The application spaces that require the uses of HSI and MSI continue to grow in number. Remote sensing, aerial imaging of the earth’s surface with the use of unmanned aerial vehicles (UAVs) and satellites, has relied on both HSI and MSI for decades. Spectral photography can penetrate through Earth’s atmosphere and different cloud cover for an unobscured view of the ground below. This technology can be used to monitor changes in population, observe geological transformations, and study archeological sites. In addition, HSI and MSI technologies have become increasingly critical in the study of the environment. Data can be collected about deforestation, ecosystem degradation, carbon recycling, and increasingly erratic weather patterns. Researchers use the information gathered to create predictive models of the global ecology, which drives many environmental initiatives meant to combat the negative effects of climate change and human influence on nature. 6
What is the spectrum of light used in machine vision?
In typical machine vision applications, illumination used and captured by the sensor is in the visible spectrum . This part of the spectrum consists of the only light that the human eye can detect, ranging from roughly 400nm (violet) to 700nm (dark red) (Figure 1). Imaging lens assemblies and sensors typically have peak spectral sensitivities around 550nm. The quantum efficiency of a camera sensor is the ability to convert photons into an electric signal; this efficiency decreases significantly into the ultraviolet or the near infrared. In the simplest terms, hyperspectral imaging (HSI) is a method for capturing images that contain information from a broader portion of the electromagnetic spectrum. This portion can start with UV light, extend through the visible spectrum, and end in the near or short-wave infrared. This extended wavelength range can reveal properties of material composition that are not otherwise apparent.
What is HSI spectroscopy?
For this reason, HSI is often referred to as imaging spectroscopy. 1. As a quick aside, a spectrometer collects wavelength information as well as relative intensity information for the different wavelengths detected. 2 These devices typically collect light from a singular source or location on a sample.
What is a spectrometer used for?
A spectrometer can be used to detect substances that scatter and reflect specific wavelengths, or material composition based on fluorescent or phosphorescent emissions. An HSI system takes this technology to the next level by assigning positional data to the collected light spectrums.
Abstract
Due to the high-dimension characteristics of hyperspectral data, dimensionality reduction is becoming an important problem in hyperspectral image classification. Band selection can retain the information which is capable of keeping the original physical meaning of the spectral channels, and thus it has attracted more research interests.
1. Introduction
Recent advances in remote sensing technology have made hyperspectral images (HSI) more widely available, with dense sampling of hundreds of narrow contiguous bands (on the order of 10 nm). This high spectral resolution provides potential for better discrimination of land covers, while also yielding large volumes of data.
3. Experimental results and discussions
We test our method on two well-known hyperspectral benchmark data sets, i.e., the Indian Pine data set and the Washington DC Mall data set. The Indian Pine data set is made up of a 145 × 145 pixel portion of the AVIRIS image acquired over NW Indian Pine in June 1992 [18].
4. Concluding remarks
In this study, a novel MCS-based hyperspectral band selection and classification framework is proposed and an embodiment has been presented.
Acknowledgments
The Washington DC Mall data set is obtained from the student CD-ROM attached to Prof. Landgrebe's book. The authors are also grateful to Prof. Serpico and Dr. Moser for providing us the training/test samples of the Indian Pine data set.
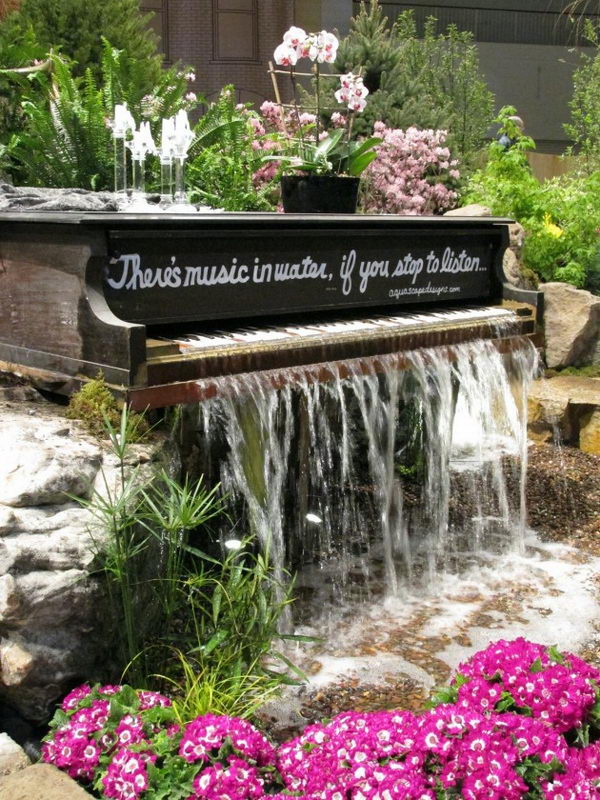