
When a trial uses a continuous measure, such as blood pressure, the treatment effect is often calculated by measuring the difference in mean improvement in blood pressure between groups. In these cases (if the data are normally distributed), a t -test is commonly used.
How do you calculate the overall treatment effect?
The sum of the regression coefficient for the treatment variable and the regression coefficient for the interaction between the treatment variable and time then reflects the overall treatment effect.
What is the effect size of a treatment?
Effect Size. An effect size is a statistical calculation that can be used to compare the efficacy of different agents by quantifying the size of the difference between treatments. It is a dimensionless measure of the difference in outcomes under two different treatment interventions.
What is the best way to measure effect size?
For categorical measures, such as “improved” versus “not improved” or “present” versus “absent,” two measures that can be used to assess effects are RR and OR. Consideration of RR is particularly useful in prospective clinical trials to assess differences in treatments.
How do you find the treatment effect at two follow-up measurements?
The overall treatment effect and the treatment effects at the two follow-up measurements can be obtained in the same way as been described for the longitudinal analysis of covariance (method 1). Table 3shows the structure of the data used to estimate the parameters of the analysis of changes.

What is a treatment effect in statistics?
Treatment effects can be estimated using social experiments, regression models, matching estimators, and instrumental variables. A 'treatment effect' is the average causal effect of a binary (0–1) variable on an outcome variable of scientific or policy interest.
How do you measure treatment effect in RCT?
To estimate a treatment effect in an RCT, the analysis has to be adjusted for the baseline value of the outcome variable. A proper adjustment is not achieved by performing a regular repeated measures analysis (method 2) or by the regular analysis of changes (method 3).
How do you determine the precise a treatment effect?
The best estimate of the treatment's effect is simply the difference in the means (or, in some trials, the medians) of the treatment and control groups.
What is a good treatment effect size?
Effect sizes of 0.8 or higher are considered large, while effect sizes of 0.5 to 0.8 can be considered moderately large. Effect sizes of less than 0.3 are small and might well have occurred without any treatment at all.
What is treatment effect Anova?
The ANOVA Model. A treatment effect is the difference between the overall, grand mean, and the mean of a cell (treatment level). Error is the difference between a score and a cell (treatment level) mean.
How do you calculate effect size in clinical trials?
This method of calculating effect sizes can be expressed mathematically as ES = (mi - m2)/sl, where m, is the pretreatment mean, m2 the posttreatment mean, and s, the pretreatment standard deviation. In this instance the before-treatment scores are used as a proxy for control group scores.
How do you appraise the effectiveness of treatment?
Three broad criteria should be assessed, including the validity of the results, the magnitude and precision of the treatment effect, and the applicability of results to patient care. Evidence-based clinical practice will lead toward higher quality patient care, and should be sought by all practicing urologists.
What is treatment effect in psychology?
the magnitude of the effect that a treatment (i.e., the independent variable) has upon the response variable (i.e., the dependent variable) in a study.
Is confidence interval the same as P value?
In exploratory studies, p-values enable the recognition of any statistically noteworthy findings. Confidence intervals provide information about a range in which the true value lies with a certain degree of probability, as well as about the direction and strength of the demonstrated effect.
Is an effect size of 0.8 good?
The larger the effect size, the larger the difference between the average individual in each group. In general, a d of 0.2 or smaller is considered to be a small effect size, a d of around 0.5 is considered to be a medium effect size, and a d of 0.8 or larger is considered to be a large effect size.
What does an effect size of .1 mean?
A value closer to -1 or 1 indicates a higher effect size. Pearson's r also tells you something about the direction of the relationship: A positive value (e.g., 0.7) means both variables either increase or decrease together.
Is effect size the same as treatment effect?
When the meta-analysis looks at the relationship between two variables or the difference between two groups, its index can be called an “Effect size”. When the relationship or the grouping is based on a deliberate intervention, its index can also be called a “Treatment effect”.
What are the advantages and disadvantages of risk measures?
Advantages and disadvantages of risk measures. Both absolute risk and relative risk measures have their advantages and disadvantages. Relative risk measures have the advantage of being stable across populations with different baseline risks and are, for instance, useful when combining the results of different trials in a meta-analysis.
What is evidence based medicine?
Evidence based medicine implies that healthcare professionals are expected to base their practice on the best available evidence. This means that we should acquire the necessary skills for appraising the medical literature, including the ability to understand and interpret the results of published articles. This article discusses in a simple, practical, ‘non-statistician’ fashion some of the important outcome measures used to report clinical trials comparing different treatments or interventions. Absolute and relative risk measures are explained, and their merits and demerits discussed. The article aims to encourage healthcare professionals to appreciate the use and misuse of these outcome measures and to empower them to calculate these measures themselves when, as is frequently the case, the authors of some original articles fail to present their results in a more clinically friendly format.
Why is absolute risk measure important?
Absolute risk measures are of immense importance in clinical practice because the reciprocal of the ARR is equivalent to the number needed to treat (NNT), which is a more user friendly way of reporting outcomes.
What is clinical trial?
clinical trial. evidence based medicine. number needed to treat. risk measures. In clinical trials comparing different interventions, outcomes can be measured in a variety of ways. Not all of these outcome measures depict the significance or otherwise of the intervention being studied in a clinically useful way.
Is 80% reduction in risk to 0.001% trivial?
However, because the baseline risk of dying (0.005%) is so trivial, the 80% reduction in risk to 0.001% is also trivial and is unlikely to be of much clinical benefit to the patient.
What is the type of error where we wrongly accept the null hypothesis of no treatment effect?
Similarly, even if we can not exclude chance as the explanation of the result from our study, it does not necessarily mean that the treatment is ineffective. This type of error—a false negative result—where we wrongly accept the null hypothesis of no treatment effect is called a type II error .
What is the SE of a study?
The SE is regarded as the unit that measures the likelihood that the result is not because of chance.
What is a chi squared test?
When a study measures categorical variables and expresses results as proportions (eg, numbers infected or wounds healed), then a χ 2 (chi-squared) test is used. This tests the extent to which the difference between the observed proportion in the treatment group is different from what would have been expected by chance if there was no real difference between the treatment and control groups. Alternatively, if the odds ratio is used, the standard error of the odds ratio can be calculated and, assuming a normal distribution, 95% confidence intervals can be calculated and hypothesis tests can be done.
Is a treatment effect statistically significant?
However, just because a test shows a treatment effect to be statistically significant, it does not mean that the result is clinically important. For example, if a study is very large (and therefore has a small standard error), it is easier to find small and clinically unimportant treatment effects to be statistically significant. A large randomised controlled trial compared rehospitalisations in patients receiving a new heart drug with patients receiving usual care. A 1% reduction in rehospitalisation was reported in the treatment group (49% rehospitalisations v 50% in the usual care group). This was highly statistically significant (p<0.0001) mainly because this is a large trial. However, it is unlikely that clinical practice would be changed on the basis of such a small reduction in hospitalisation.
Can we measure average treatment effects?
Unfortunately, as a result of the fundamental problem of causal inference, we cannot directly measure average treatment effects. This is because we cannot witness more than one potential outcome, as we cannot set an explanatory variable to more than one value.
Does UI have the same effect on all people?
Often times, a policy solution, UI feature, or medical therapy does not have the same effect on all individuals in a population; causal inference often involves estimating treatment responses despite these differences.
Measure everything!
No. Quite the contrary. Clinicians should aim to properly select measures that are relevant to the patient: main complaint, goals, condition, and/or diagnosis (if one exists). In addition, the measures chosen should be sufficiently responsive to change, encompass multiple constructs, and cross domains.
Measure nothing, clinical outcomes are meaningless!
No. Quite the contrary. In addition, to selecting appropriate outcomes measurements, clinicians must integrate and understand appropriate current clinical, mechanistic, and basic science research.
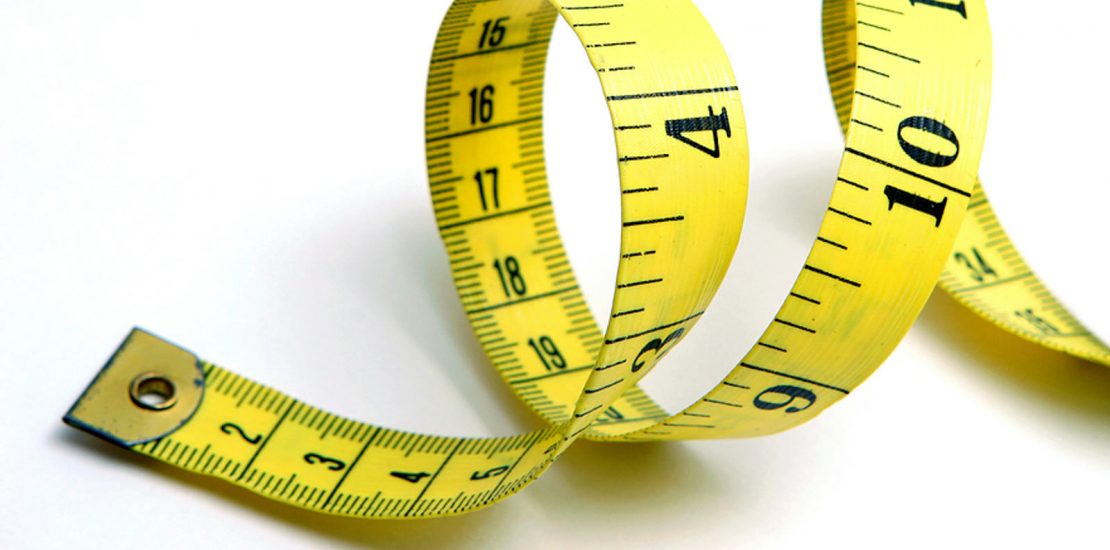