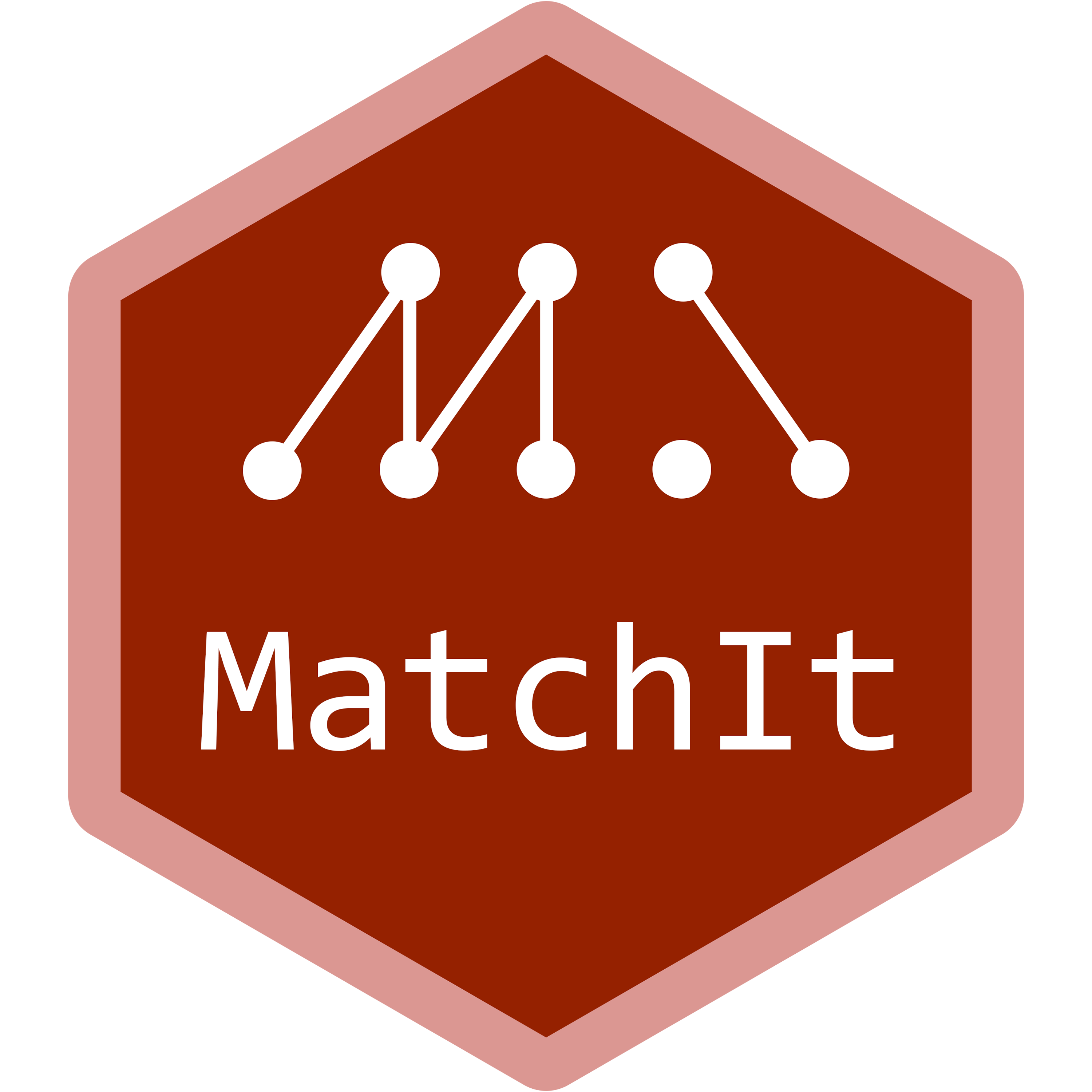
Like regression, matching estimators identify treatment effects through balancing observed covariates across treatment groups.
Full Answer
What is the most common estimand in matching?
Different matching methods allow you to estimate effects that can generalize to different target populations. The most common estimand in matching the average treatment effect in the treated (ATT), which is the average effect of treatment for those who receive treatment.
How is the effect of the treatment estimated in the matched sample?
After assessing balance and deciding on a matching specification, it comes time to estimate the effect of the treatment in the matched sample. How the effect is estimated and interpreted depends on the desired estimand and the type of model used (if any).
How do you estimate the effect in the matched dataset?
In general, whether a caliper, common support restriction, exact matching specification, or k :1 matching specification is used, estimating the effect in the matched dataset (i.e., the output of match.data ()) is straightforward and involves fitting a model for the outcome that incorporates the matching weights 3.
How do you estimate effects after full matching?
Full matching presents fairly straightforward methods of effect and standard error estimation. The most common and recommended way to estimate effects after full matching is to use the computed matching weights to estimate weighted effects.

How do you estimate the treatment effect?
CONTINUOUS MEASURES When a trial uses a continuous measure, such as blood pressure, the treatment effect is often calculated by measuring the difference in mean improvement in blood pressure between groups. In these cases (if the data are normally distributed), a t-test is commonly used.
What is matching estimation?
Matching estimator has been widely used across disciplines such as statistics, economics, sociology, political science, etc, to estimate causal effects. It is a quasi-experimental method that aims to search for counterfactual unit that is comparable with the treated unit among many untreated units.
How do you evaluate matching?
The evaluation of the matching can be measured by finding Correlation (to be more accurate cross correlation) between the image and the template or Sum of Squared Error (SSE) . If you program with Matlab , you can use their template matching functions.
What are treatment effects in statistics?
A 'treatment effect' is the average causal effect of a binary (0–1) variable on an outcome variable of scientific or policy interest.
What is matching in data analysis?
Matching is a statistical technique which is used to evaluate the effect of a treatment by comparing the treated and the non-treated units in an observational study or quasi-experiment (i.e. when the treatment is not randomly assigned).
Is matching better than regression?
Matching is a way to discard some data so that the regression model can fit better. Trying to do matching without regression is a fool's errand or a mug's game or whatever you want to call it.
What is matching in impact evaluation?
Describes matching as an impact evaluation method that uses large data sets and statistical techniques to construct an artificial comparison group and which can apply in the context of almost any program assignment rules, as long as a group exists that has not participated in the program.
What are the common matching methods that can be used in matching rules?
Required EditionsMatching MethodMatching AlgorithmsScoring MethodFuzzy: PhoneExactWeighted AverageFuzzy: CityEdit Distance ExactMaximumFuzzy: StreetExactWeighted AverageFuzzy: ZIPExactWeighted Average5 more rows
What is the optimal matching model?
Optimal matching is a sequence analysis method used in social science, to assess the dissimilarity of ordered arrays of tokens that usually represent a time-ordered sequence of socio-economic states two individuals have experienced.
Is effect size the same as treatment effect?
When the meta-analysis looks at the relationship between two variables or the difference between two groups, its index can be called an “Effect size”. When the relationship or the grouping is based on a deliberate intervention, its index can also be called a “Treatment effect”.
What is the treatment effect in Anova?
The ANOVA Model. A treatment effect is the difference between the overall, grand mean, and the mean of a cell (treatment level). Error is the difference between a score and a cell (treatment level) mean.
Why do analysts randomly assign treatment?
In experimental studies, or studies where an analyst has control over treatment assignment, analysts can randomly assign treatment to individuals to ensure that the treatment and the potential outcomes of observed individuals are drawn from independent probability distributions. For example, if I were to assign email alerts with images to my email subscribers randomly, their potential outcomes from receiving emails with and without images, would be statistically independent from the event that they were chosen for treatment. While randomizing treatment enables the SDO to be an unbiased estimate of ATE, in order to minimize the variance of the estimate practitioners must also ensure they are calculating an SDO from a sufficiently large sample population.
Can we measure average treatment effects?
Unfortunately, as a result of the fundamental problem of causal inference, we cannot directly measure average treatment effects. This is because we cannot witness more than one potential outcome, as we cannot set an explanatory variable to more than one value.
Does UI have the same effect on all people?
Often times, a policy solution, UI feature, or medical therapy does not have the same effect on all individuals in a population; causal inference often involves estimating treatment responses despite these differences.
Is SDO the same as ATE?
It is the intuitive estimation strategy you may be familiar with, simply the difference in means between treated and untreated individuals. Unfortunately, it is not exactly equivalent to the ATE. The SDO has two main sources of bias which can systematically distort the statistic away from the true value of an average treatment effect. The equation relating the SDO to the average treatment effect is as follows:
Why should treatment choices not be made based on comparisons of statistical significance?
When the results of clinical trials are statistically significant, treatment choices should not be made based on comparisons of statistical significance, because the magnitude of statistical significance is heavily influenced by the number of patients studied. Therefore, a small trial of a highly effective therapy could have a statistically significant result that is smaller than a result from a large trial of a modestly effective treatment.
What is the SMD measure of effect?
The standardized mean difference (SMD) measure of effect is used when studies report efficacy in terms of a continuous measurement, such as a score on a pain-intensity rating scale. The SMD is also known as Cohen’s d.5
What does a SMD of zero mean?
An SMD of zero means that the new treatment and the placebo have equivalent effects. If improvement is associated with higher scores on the outcome measure, SMDs greater than zero indicate the degree to which treatment is more efficacious than placebo, and SMDs less than zero indicate the degree to which treatment is less efficacious than placebo. If improvement is associated with lower scores on the outcome measure, SMDs lower than zero indicate the degree to which treatment is more efficacious than placebo and SMDs greater than zero indicate the degree to which treatment is less efficacious than placebo.
How to calculate POB statistic?
For binary variables, the POB statistic can be computed from the absolute difference (AD) in treatment response as follows: POB = 0.5(AD+1).
How many meta-analyses did Song et al.2examine?
Song et al.2examined 44 published meta-analyses that used a measure of effect magnitude to compare treatments indirectly. In most cases, results obtained by indirect comparisons did not differ from results obtained by direct comparisons. However, for three of the 44 comparisons, there were significant differences between the direct and the indirect estimates.
Why are indirect comparisons important?
When indirect comparisons are conducted, measures of effect magnitude are essential in order to make sensible evaluations. For example, if one study measured the efficacy of a therapy for back pain using a five-point rating scale for pain intensity and another study used a 10-point rating scale, we could not compare the results, because a one-point decrease has a different meaning for each scale. Even if two studies use the same measure, we cannot simply compare changed scores between treatment and placebo, because these studies may differ in their standards for precision of measurement. These problems of differing scales of measurement and differences in precision of measurement make it difficult to compare studies. Fortunately, these problems can be overcome if we use estimates of effect magnitude, which provide the difference in improvement between therapy and placebo, adjusted for the problems that make the statistical significance level a poor indicator of treatment efficacy.
How should health care professionals choose among the many therapies claimed to be efficacious for treating specific disorders?
Ideally, health care professionals would compare different treatments by referring to randomized, double-blind, head-to-head trials that compared the treatment options. Although individual medications are typically well researched when these placebo-controlled studies are performed, studies that directly compare treatments are rare. In the absence of direct head-to-head trials, other evidence comes from indirect comparisons of two or more therapies by examining individual studies involving each treatment.
