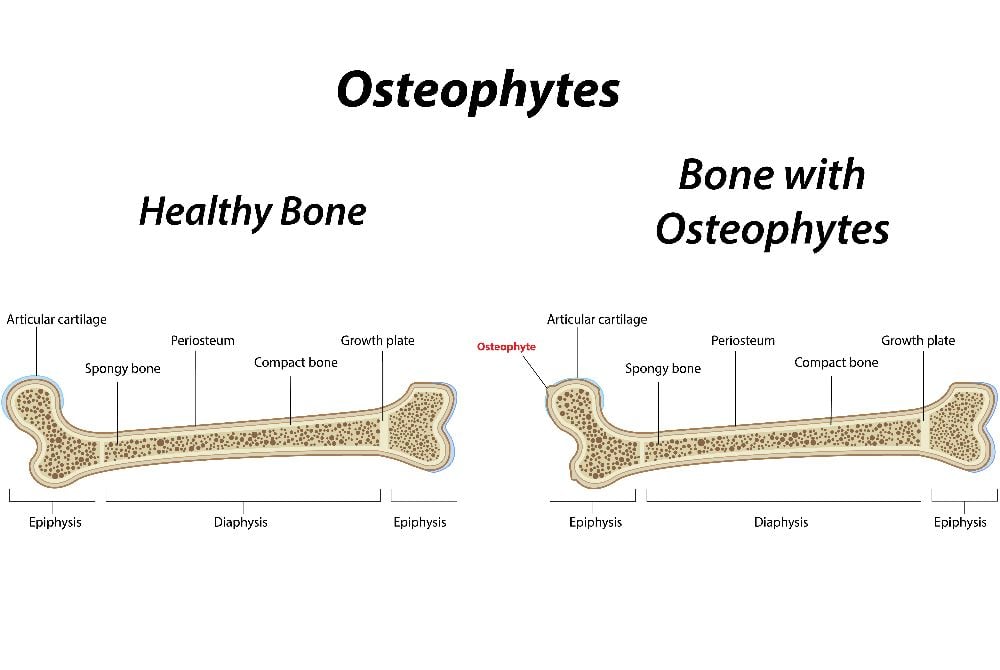
Some researchers call a treatment effect "heterogenous" if it affects different individuals differently (heterogeneously). For example, perhaps the above treatment of a job search monitoring policy affected men and women differently, or people who live in different states differently.
Full Answer
How can I compare two groups of patients with different treatments?
The change in the outcome variable in the treatment group compared to the change in the outcome in the control group gives a measure for the treatment effect. The difference in a given outcome of the project, between the treated members and the control group, can be computed before the treatment is implemented. This difference is usually referred to as the “first …
How do you calculate difference in differences between treatment and timing?
· Difference-in-Differences Methodology. Sep 25, 2019 26 min read. Introduction. In this methodological section I will explain the issues with difference-in-differences (DiD) designs when there are multiple units and more than two time periods, and also the particular issues that arise when the treatment is conducted at staggered periods in time.
Can the effects of treatment be heterogeneous?
· Finally, in Figure 18.4 (d), any remaining before/after difference in the treated (California) group is the difference-in-difference effect. The raw difference is 26.3% − 27.1%, …
Is there something statistically unusual about the actual treatment profile?
· For any particular individual, the unobserved potential outcome is called the counterfactual. The individual's treatment effect, , is therefore never available because only …
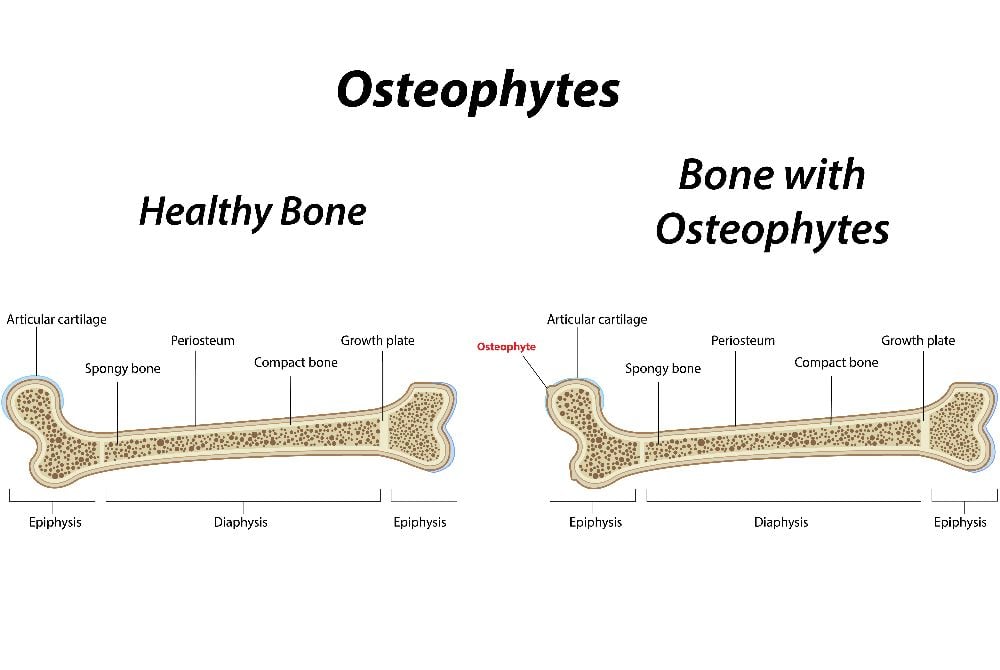
How do you explain difference in differences?
The difference-in-differences method is a quasi-experimental approach that compares the changes in outcomes over time between a population enrolled in a program (the treatment group) and a population that is not (the comparison group). It is a useful tool for data analysis.
What does difference in differences control for?
Difference-in-differences (DD) methods attempt to control for unobserved variables that bias estimates of causal effects, aided by longitudinal data collected from students, school, districts, or states.
When can difference in difference be used?
DID relies on a less strict exchangeability assumption, i.e., in absence of treatment, the unobserved differences between treatment and control groups arethe same overtime. Hence, Difference-in-difference is a useful technique to use when randomization on the individual level is not possible.
What is difference regression difference?
Difference in differences (DiD) is a non-experimental statistical technique used to estimate treatment effects by comparing the change (difference) in the differences in observed outcomes between treatment and control groups, across pre-treatment and post-treatment periods.
What is a difference in differences in research?
Difference in differences (DID or DD) is a statistical technique used in econometrics and quantitative research in the social sciences that attempts to mimic an experimental research design using observational study data, by studying the differential effect of a treatment on a 'treatment group' versus a 'control group' ...
Why does difference in difference matching work?
Difference-in-differences requires parallel trends but allows for level effect imbalance between the treatment and control group. Matching requires all confounders to be balanced between the two groups but does not require parallel trends.
Should we combine difference in differences with conditioning on pre treatment outcomes?
Taken together, these results suggest that we should not combine DID with conditioning on pre-treatment outcomes but rather use DID conditioning on covariates that are fixed over time. When the PTA fails, DID applied symmetrically around the treatment date performs well in simulations and when compared with RCTs.
What is a difference in difference design?
Abstract. The difference in difference (DID) design is a quasi-experimental research design that researchers often use to study causal relationships in public health settings where randomized controlled trials (RCTs) are infeasible or unethical.
What is a staggered difference in difference?
One difference stems from differences across counties within the same birth cohort, while the other difference stems from differences within counties across different birth cohorts (those born later are more exposed to the program than those born later).
How do you conduct a difference difference analysis?
General Method The data is analyzed by first calculating the difference in first and second time periods, and then subtracting the average gain (or difference) in the control group from the average gain (or difference) in the treatment group.
What is a generalized difference in differences?
The modified DD is a generalized difference in differences (GDD), which is a DD with one additional time-wise difference. GDD allows the selection effect to be a constant that is not necessarily zero, and the constant is removed by the additional time-wise difference using the two pretreatment periods.
What is the counterfactual in difference in difference?
Counterfactual Assumption (2b) essentially disregards time points other than these two. That is, the other time points need not satisfy any “parallel trends” assumption. While this assumption is perfectly valid if true, using such an assumption requires justification.
What is the difference in difference estimator?
The difference in difference (or "double difference") estimator is defined as the difference in average outcome in the treatment group before and after treatment minus the difference in average outcome in the control group before and after treatment3: it is literally a "difference of differences."
What is the difference between RCT and difference in difference?
The difference in difference (DID) design is a quasi-experimental research design that researchers often use to study causal relationships in public health settings where randomized controlled trials (RCTs) are infeasible or unethi- cal. However, causal inference poses many challenges in DID designs.
How do you conduct a difference difference analysis?
General Method The data is analyzed by first calculating the difference in first and second time periods, and then subtracting the average gain (or difference) in the control group from the average gain (or difference) in the treatment group.
What does difference mean in math terms?
To find the difference between two numbers, subtract the number with the smallest value from the number with the largest value. The product of this sum is the difference between the two numbers. For instance, this is how you would find the difference between 45 and 100: 100 - 45 = 55.
When the treatment effects do not change over time, what is the variance weighted average of cross-group treatment effects
Furthermore, GB (2019) shows that when the treatment effects do not change over time, β D D β D D is the variance-weighted average of cross-group treatment effects, and all of the weights are positive. However, when the treatment effect does vary across time, some of these 2x2 estimates enter the average with negative weights. This is because already-treated units act as controls, and changes in a portion of their treatment effects over time are subtracted from the DiD estimate.
What is the parallel trend assumption that the two units would have the same change in the outcome variable?
Under the parallel trends assumption that, ceteris paribus, the two units would have the same change in the outcome variable, the treatment effect is equal to the difference in the outcome variable for the treated unit in period 2 ( Y T 2 Y 2 T) and the treated unit in period 1 ( Y T 1 Y 1 T ), less the same difference in the control unit ( Y C 2 − Y C 1 Y 2 C − Y 1 C ).
What is the general estimator from the TWFE approach?
According to GB (2019), the general estimator from the TWFE approach is actually a “weighted average of all possible two-group/two-period DiD estimators in the data.”
What is VWCT in statistics?
VWCT is the “variance-weighted common trend” term, which generalizes the common trend assumption of DiD to a setting with timing variation . VWCT is the average of the difference in counterfactural trends between pairs of groups and different time periods using the weights from the previous decomposition, and captures how differential trends map to bias in the ^ β D D β ^ D D estimate. Essentially, this captures the fact that different groups might not have the same underlying trend in outcome dynamics, which biases the (really any) DiD estimate.
What is the TWFE approach?
Under the TWFE approach, researchers estimate the regression with dummies for cross-sectional units ( α i α i) and time periods ( α t α t) and a treatment dummy ( D i t D i t ). The POST and TREAT variables are thus subsumed by the fixed effects structure. This regression is generally written as:
Does panel length change the DiD estimate?
What is clear from the derivation is that panel length alone can change the DiD estimates substantially, even when each 2x2 DiD estimate is constant. This seems normatively undesirable. In addition, the weights assigned to each 2x2 estimate when aggregating through TWFE OLS are a result of the size of the subsample and the magnitude of the treatment variance. Groups treated closer to the middle of the panel get more weight, which again isn’t intuitively desirable.
When was the difference in difference technique invented?
The difference-in-difference (DID) technique originated in the field of econometrics, but the logic underlying the technique has been used as early as the 1850’s by John Snow and is called the ‘controlled before-and-after study’ in some social sciences.
When to examine composition of population in treatment/intervention and control groups?
Be sure to examine composition of population in treatment/intervention and control groups before and after intervention
Which groups have parallel trends in outcome?
Treatment/intervention and control groups have Parallel Trends in outcome (see below for details)
What is a DID?
DID is a quasi-experimental design that makes use of longitudinal data from treatment and control groups to obtain an appropriate counterfactual to estimate a causal effect. DID is typically used to estimate the effect of a specific intervention or treatment (such as a passage of law, enactment of policy, or large-scale program implementation) ...
Can difference in differences be modified?
We can do that! Difference-in-differences can be modified just a bit to allow the effect to differ in each time period. In other words, we can have dynamic treatment effects. This lets you see things like the effect taking a while to work, or fading out. 508 508 You’ll notice many similarities to Chapter 17, and indeed this is often referred to as "event study difference-in-differences.’’
Is there a problem with two way fixed effects regression?
What’s the problem? Well, from a research design perspective, there’s no problem. You’re just tossing a bunch of valid difference-in-difference designs together. But from a statistical perspective, this makes our two-way fixed effects regression not work any more ( Goodman-Bacon 2018). The problem can actually be so bad that, in some rare cases, you can get a negative difference-in-differences estimate even if the true effect is positive for everyone in the sample. And thus the secret shame: for decades researchers were basically unaware of this problem and used two-way fixed effects anyway. Only very recently is the tide beginning to turn on this.
Is difference in differences an assumption?
This assumption, though it’s completely crucial for what we’re doing with difference-in-differences, must remain an assumption. It relies directly on a counterfactual observation - what would have happened without the treatment.
Can the difference between treated and untreated groups change from before treatment to after treatment?
The parallel trends assumption says that, if no treatment had in fact occurred, then the difference in outcomes between the treated and untreated groups would not have changed from before the treatment date to afterwards. It’s okay that there is a difference, 504 504 Although one does wonder whether we have a good comparison group if the outcomes are very different. but that difference can’t change from before treatment to after treatment for any reason but treatment.
How to distinguish between untreated and treated outcomes?
1 To clearly distinguish between treated and untreated potential outcomes, we use a potential outcomes notation where , , are observed outcomes for the treated group (but is not an observed outcome for the treated group) and , , and are observed outcomes for the untreated group.
What is the fundamental problem of treating effects literature?
The fundamental problem is that exactly one (never both) of these outcomes is observed for a particular individual. Using the above notation, the observed outcome can be expressed as follows:
What is the distributional difference in differences assumption?
The Distributional Difference in Differences Assumption says that the distribution of the change in untreated potential outcomes does not depend on whether or not the individual belongs to the treated or the untreated group. Intuitively, it generalizes the idea of “parallel trends” holding on average to the entire distribution. In applied work, the validity of using a difference in differences approach to estimate the ATT hinges on whether the unobserved trend for the treated group can be replaced with the observed trend for the untreated group. This is exactly the same sort of thought experiment that needs to be satisfied for the Distributional Difference in Differences Assumption to hold. Being able to invoke a standard assumption to identify the QTT stands in contrast to the existing literature on identifying the QTT in similar models which generally require less familiar assumptions on the relationship between observed and unobserved outcomes.
Why is the QTT only partially identified?
Even under this Distributional Difference in Differences Assumption, the QTT is still only partially identified because it depends on the unknown dependence between the change in untreated potential outcomes and the initial level of untreated potential outcomes for the treated group. We introduced the Copula Stability Assumption which says that the missing dependence is constant over time. Under this assumption and when panel data is available, the QTT is point identified. We show that the Copula Stability Assumption is likely to hold in exactly the type of models that are typically estimated using difference in differences techniques under mild additional conditions. This idea of a time invariant copula may also be valuable in other areas of microeconometric research especially when a researcher has access to panel data.
How to estimate counterfactual quantiles?
We estimate counterfactual quantiles by where which follows from the identification result in Theorem 1 and where distribution functions are estimated by empirical distribution functions and quantile functions are estimated by inverting empirical distribution functions; here, is the number of observations in the treated group and is the set of treated individuals.
What is parallel trend?
It states that, on average, the unobserved change in untreated potential outcomes for the treated group is equal to the observed change in untreated outcomes for the untreated group. To study the QTT, Assumption 3.1 needs to be strengthened because the QTT depends on the entire distribution of untreated outcomes for the treated group rather than only the mean of this distribution.
Why do the second and fourth equalities hold?
where the second and fourth equalities hold because and the third equality holds because the marginal distribution of time varying unobservables does not change over time. This result implies that both for the treated group and untreated group the average change in untreated potential outcomes is 0 which implies the claim.
What is the treatment effect?
A treatment effect that differs from individual to individual. Intent-to-Treat. The average treatment effect of assigning treatment, in a context where not everyone who is assigned to receive treatment receives it (and maybe some people not assigned to treatment get it anyway). Local Average Treatment Effect.
What is the mean of the treatment effect distribution?
The mean of the treatment effect distribution is called, for reasons that should be pretty obvious, the average treatment effect. The average treatment effect , often referred to as the ATE, is in many cases what we’d like to estimate.
Is a treatment group the same as a treatment group?
The treatment groups are not the same. An individual can belong one, both or neither of the treatment groups. If one belongs to the first treatment group it is likely that one also belongs to the second group. Both treatments are permanent.
Does intercept make sense?
0. Yes it make sense. You should include also an intercept term (unless you have good reason to not do so) which is excluded from your formula. Individuals who do not belong to treatment group 1 or 2 will form the reference (or control) group. If a large group of individuals belong to both groups, it might be difficult to separate B 1 and B 2 ...
What is difference in differences design?
The difference-in-differences design is an early quasi-experimental identification strategy for estimating causal effects that predates the randomized experiment by roughly eighty-five years. It has become the single most popular research design in the quantitative social sciences, and as such, it merits careful study by researchers everywhere. 138 In this chapter, I will explain this popular and important research design both in its simplest form, where a group of units is treated at the same time, and the more common form, where groups of units are treated at different points in time. My focus will be on the identifying assumptions needed for estimating treatment effects, including several practical tests and robustness exercises commonly performed, and I will point you to some of the work on difference-in-differences design (DD) being done at the frontier of research. I have included several replication exercises as well.
What is inside the DD parameter estimate when using twoway fixed effects?
Up to now, we just showed what was inside the DD parameter estimate when using twoway fixed effects: it was nothing more than an “adding up” of all possible 2 × 2 s weighted by group shares and treatment variance. But that only tells us what DD is numerically; it does not tell us whether the parameter estimate maps onto a meaningful average treatment effect. To do that, we need to take those sample averages and then use the switching equations replace them with potential outcomes. This is key to moving from numbers to estimates of causal effects.
Who evaluated the impact that a gun reform had on violence and to illustrate various principles and practices regarding differential timing?
Cheng and Hoekstra ( 2013) evaluated the impact that a gun reform had on violence and to illustrate various principles and practices regarding differential timing. I’d like to discuss those principles in the context of this paper. This next section will discuss, extend, and replicate various parts of this study.
Did Snow calculate the difference in differences?
While Snow did not explicitly calculate the difference-in-differences, the ability to do so was there ( Coleman 2019). If we difference Lambeth’s 1854 value from its 1849 value, followed by the same after and before differencing for Southwark and Vauxhall, we can calculate an estimate of the ATT equaling 78 fewer deaths per 10,000. While Snow would go on to produce evidence showing cholera deaths were concentrated around a pump on Broad Street contaminated with cholera, he allegedly considered the simple difference-in-differences the more convincing test of his hypothesis.
What are the different types of physical differences?
Types of Individual Differences: 1. Physical differences: Shortness or tallness of stature, darkness or fairness of complexion, fatness, thinness, or weakness are various physical individual differences. 2.
What are the differences in attitudes?
Differences in attitudes: Individuals differ in their attitudes towards different people, objects, institutions and authority. 4. Differences in achievement: It has been found through achievement tests that individuals differ in their achievement abilities.
Why are there differences in achievement?
These differences in achievement are even visible among the children who are at the same level of intelligence. These differences are on account of the differences in the various factors of intelligence and the differences in the various experiences, interests and educational background.
Why do people differ in their intellectual and intellectual development?
Physical, intellectual and emotional development is caused by the growth in age. Many individuals differ because of the differences in intelligence. Individuals who are below the average in intelligence and mental age find much difficulty in learning and the average intelligent persons can learn quickly.
Why do boys and girls have different development?
Development of boys and girls exhibits differences due to difference in sex. The physical development of the girl takes place a year or two earlier than the boys. Between the age of 11 and 14, girls are taller and heavier than the boys. After 15, boys start winning the race.
How do different nations differ?
Individuals of different nations differ in respect of physical and mental differences, interests and personality etc. ‘Russians are tall and stout’; ‘Ceylonese are short and slim’; ‘Germans have no sense of humour’; ‘Yellow races are cruel and revengeful’; ‘Americans are hearty and frank’; Indians are timid and peace-loving’ and the like observations enter into our common talk.
Who discovered the differences between men and women?
McNemar and Terman discovered the following differences between men and women, on the basis of some studies:
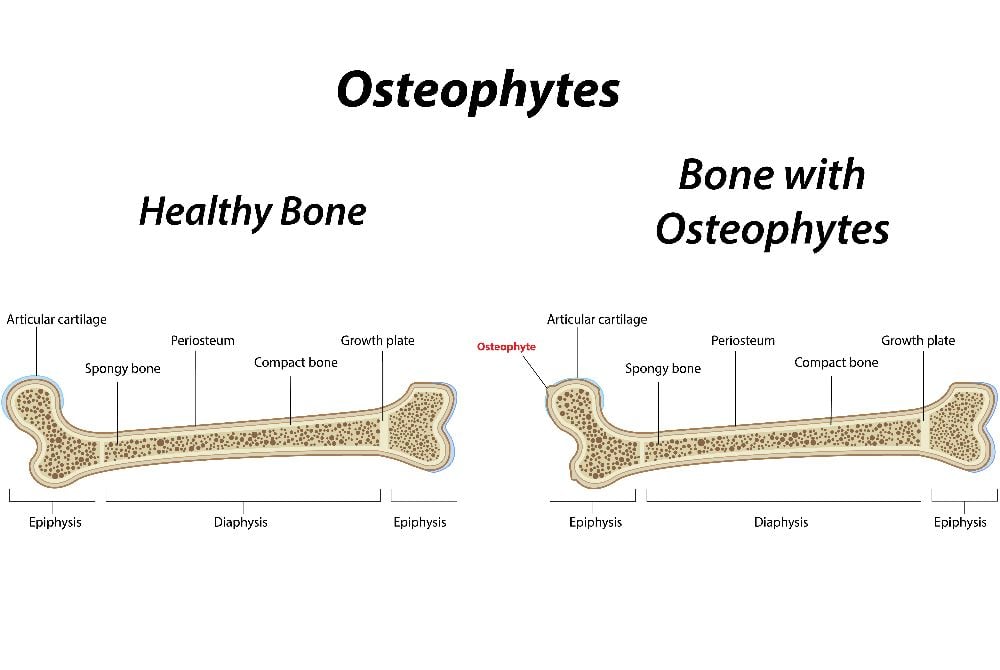