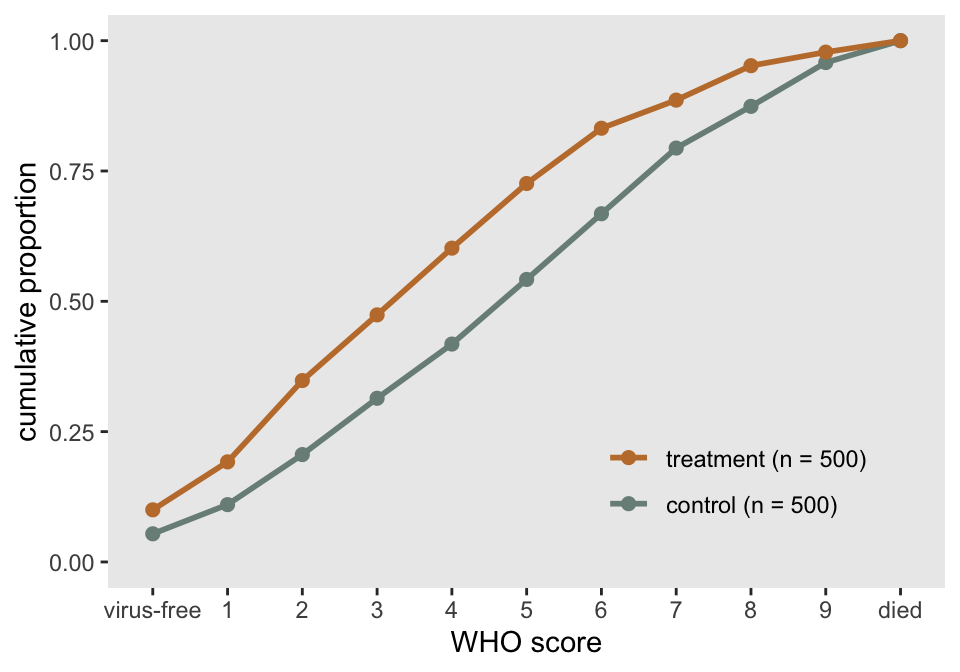
Estimating the Size of Treatment Effects
- Cohen’s d. Cohen’s d is used when studies report efficacy in terms of a continuous measurement, such as a score on a...
- Relative Risk (RR). Cohen’s d is useful for estimating effect sizes from quantitative or dimensional measures. For...
- Odds Ratio (OR). While RR is an appropriate measure for prospective studies, such as...
Full Answer
How do you find the effect size of a treatment?
Abstract. In randomized clinical trails (RCTs), effect sizes seen in earlier studies guide both the choice of the effect size that sets the appropriate threshold of clinical significance and the rationale to believe that the true effect size is above that threshold worth pursuing in an RCT. That threshold is used to determine the necessary ...
What determines the size of effect in randomized clinical trials?
· In a clinical evaluation, the greater the treatment effect (expressed as the number of SEs away from zero), the more likely it is that the null hypothesis of zero effect is not …
How do you calculate the treatment effect in a clinical trial?
The effects of violating the assumptions of the instrumental variables analysis were also assessed. Sample sizes of up to 200,000 patients were considered. Results: Two-stage least …
How can we estimate the effect of treatment on patient stratification?
· For binary outcome measures in RCTs (success/failure), the most common effect size in current use is the odds ratio (OR) (Grissom and Kim 2005). If the success rate in T is s T …

How do you know how large a treatment effect?
When a trial uses a continuous measure, such as blood pressure, the treatment effect is often calculated by measuring the difference in mean improvement in blood pressure between groups. In these cases (if the data are normally distributed), a t-test is commonly used.
What does a large treatment effect mean?
Effect Size An estimate of how large the treatment effect is, that is how well the intervention worked in the. experimental group in comparison to the control. group. The larger the effect size, the stronger are the.
How do you analyze treatment effects?
The basic way to identify treatment effect is to compare the average difference between the treatment and control (i.e., untreated) groups. For this to work, the treatment should determine which potential response is realized, but should otherwise be unrelated to the potential responses.
Is a larger effect size better?
In social sciences research outside of physics, it is more common to report an effect size than a gain. An effect size is a measure of how important a difference is: large effect sizes mean the difference is important; small effect sizes mean the difference is unimportant.
What is a significant SMD?
SMD values of 0.2-0.5 are considered small, values of 0.5-0.8 are considered medium, and values > 0.8 are considered large. In psychopharmacology studies that compare independent groups, SMDs that are statistically significant are almost always in the small to medium range.
What is treatment effect Anova?
The ANOVA Model. A treatment effect is the difference between the overall, grand mean, and the mean of a cell (treatment level). Error is the difference between a score and a cell (treatment level) mean.
What is the average treatment effect on the treated?
The average treatment effect (ATE) is a measure used to compare treatments (or interventions) in randomized experiments, evaluation of policy interventions, and medical trials. The ATE measures the difference in mean (average) outcomes between units assigned to the treatment and units assigned to the control.
How precise was the treatment effect?
The best estimate of the size of the treatment effect (70 per cent) and the 95 per cent confidence interval about this estimate (7 to 100 per cent) are shown. The best estimate of the treatment effect is that it is clinically worthwhile, but this conclusion is subject to a high degree of uncertainty.
Why are trials stopped early?
However, early termination may introduce bias secondary to chance deviations from the “true effect” of treatment which would decrease if the trial was continued to completion. [15] Small trials and those with few outcome events are particularly prone to this bias if stopped early.[2] For this reason, critical readers of the urology literature should interpret trials terminated early with caution. In the case of the REDUCE trial, it appears that the trial went to completion, so this is not a concern in terms of the validity of the trial.
What is the validity of clinical trials?
Validity of clinical trials hinges upon balancing patient prognosis at the initiation, execution, and conclusion of the trial. Readers should be aware of not only the magnitude of the estimated treatment effect, but also its precision. Finally, urologists should consider all patient-important outcomes as well as the balance of potential benefits, harms, and costs, and patient values and preferences when making treatment decisions.
Why is follow up important at the end of a trial?
In order to assure that both experimental and control groups are balanced at the end of a trial, complete follow-up information on each patient enrolled is important. Unfortunately, this is rarely the case at the close of a trial. Therefore, it is important to understand to what extent follow-up was incomplete.
Did the Reduce trial blind patients?
In the REDUCE trial,[6] several important groups were blinded. The control group received a placebo, so patients should have been blind to treatment allocation. Although not explicitly stated, it appears that clinicians were blinded, as the authors describe efforts to prevent unblinding by adjusting the PSA level of those patients on dutasteride to compensate for the expected reduction in this marker. The central pathology assessors were blinded to treatment allocation. It is unclear whether data collectors or analysts were blinded to treatment allocation. Overall, however, it does appear that the investigators made a reasonable attempt to blind important groups within the conduct of this trial.
Why is blinding important in clinical trials?
Blinding is important to maintaining prognostic balance as the study progresses, as it helps to minimize a variety of biases, such as placebo effects or co-interventions. Empirical evidence of bias exists in trials where blinding was not utilized or was ineffective.[10,11] Five important groups should be blinded, when feasible: patients, clinicians, data collectors, outcome adjudicators, and data analysts [Table 1]. Frequently readers will see the terms “double-blind” or “triple-blind.” These terms may be confusing, and it is preferable to state exactly which groups are blinded in the course of a trial.[12] In surgical trials it is often impossible to blind the surgeon, but it may be feasible to blind patients, and is almost always feasible to blind data collectors and outcome assessors.
What is concealment of randomization?
Concealment of randomization is another important concept in assuring that patients entering a trial share a similar prognosis. Essentially, concealment means that study personnel who enroll patients cannot predict the group assignment (experimental or control) of the next subject. Awareness of the allocation for the next subject may consciously or unconsciously influence an investigators decision to enroll a particular patient in the trial. Lack of concealment, or poor reporting of concealment, has been empirically associated with bias in RCTs.[9] In the REDUCE trial, whether and how randomization was concealed is not explicitly reported. In a large, multicenter trial, concealment is frequently accomplished by the use of a centralized randomization center.
What is the purpose of randomization?
The purpose of randomization is to balance both known and unknown prognostic factors between control and experimental groups. When successful, randomization assures us that the only prognostic difference between experimental and control groups is the treatment under investigation, and thus, any observed effect of therapy is due to that treatment.
When a study is undertaken, the number of patients should be sufficient to allow the study to have enough power to reject
When a study is undertaken, the number of patients should be sufficient to allow the study to have enough power to reject the null hypothesis if a treatment effect of clinical importance exists. Researchers should, therefore, carry out a power or sample size calculation when designing a study to ensure that it has a reasonable chance of correctly rejecting the null hypothesis. This prior power calculation should be reported in the paper.
Why is it possible to see a benefit or harm in a clinical trial?
It is possible that a study result showing benefit or harm for an intervention is because of chance, particularly if the study has a small size. Therefore, when we analyse the results of a study, we want to see the extent to which they are likely to have occurred by chance. If the results are highly unlikely to have occurred by chance, we accept that the findings reflect a real treatment effect.
What is the problem with clinical research?
One of the problems in clinical research is the plethora of studies that are too small and so have insufficient power. In these cases, one cannot interpret a statistically non-significant result to mean that no treatment effect exists.
What happens if a study is too small?
If a study is too small, the confidence intervals can be so wide that they cannot really exclude from the range a value indicating no effect. For example, several studies of debriding agents for the treatment of chronic wounds are so small that estimates have large confidence intervals. A study of cadexomer iodine, for example, reported an odds ratio for healed wounds of 5.5 favouring cadexomer iodine compared with dextranomer. 3 However, because only 27 patients were included in the trial, the 95% confidence interval was wide, ranging from 0.88 to 34.48. Thus, the study was too small to be able to exclude an odds ratio of 1 (no treatment difference).
What is the SE of a study?
The SE is regarded as the unit that measures the likelihood that the result is not because of chance.
What is the effect of the number of SEs away from zero?
In a clinical evaluation, the greater the treatment effect (expressed as the number of SEs away from zero), the more likely it is that the null hypothesis of zero effect is not supported and that we will accept the alternative of a true difference between the treatment and control groups. In other words, the number of SEs that the study result is away from the null value, is equivalent in the court case analogy to the amount of evidence against the innocence of the defendant. The SE is regarded as the unit that measures the likelihood that the result is not because of chance. The more SEs the result is away from the null, the less likely it is to have arisen by chance, and the more likely it is to be a true effect.
What is the difference between the sample size and sampling error?
The larger the sample (n), the smaller the sampling error.
What is effect size in RCT?
In randomized clinical trails (RCTs), effect sizes seen in earlier studies guide both the choice of the effect size that sets the appropriate threshold of clinical significance and the rationale to believe that the true effect size is above that threshold worth pursuing in an RCT. That threshold is used to determine the necessary sample size for the proposed RCT. Once the RCT is done, the data generated are used to estimate the true effect size and its confidence interval. Clinical significance is assessed by comparing the true effect size to the threshold effect size. In subsequent meta-analysis, this effect size is combined with others, ultimately to determine whether treatment (T) is clinically significantly better than control (C). Thus, effect sizes play an important role both in designing RCTs and in interpreting their results; but specifically which effect size? We review the principles of statistical significance, power, and meta-analysis, and commonly used effect sizes. The commonly used effect sizes are limited in conveying clinical significance. We recommend three equivalent effect sizes: number needed to treat, area under the receiver operating characteristic curve comparing T and C responses, and success rate difference, chosen specifically to convey clinical significance.
What is the effect size of a binary outcome measure?
For binary outcome measures in RCTs (success/failure), the most common effect size in current use is the odds ratio (OR) ( Grissom and Kim 2005 ). If the success rate in T is s T and that in C is s C, then OR = [s T / (1 − s T )]/ [s C / (1 − s C )]. Odds ratio is not scaled like the hypothetical effect size of Figure 1, but again that is of little consequence. Often used instead are the γ coefficient [γ = (OR − 1)/ (OR + 1)] or Yule’s Index [Y = (OR 1/2 − 1)/ (OR 1/2 + 1)], each of which differently rescales the OR to correspond to an effect size like that in Figure 1.
What is the AUC of a RCT?
If one sampled a T patient and a C patient, AUC is the probability that the T patient has a treatment outcome preferable to the C patient (where we toss a coin to break any ties) symbolically: AUC = probability ( T > C) + .5 probability ( T = C). Thus, if AUC = .50, the T patient outcome is as likely as not to be better than that for the C patient (i.e., no effect), and AUC = 1.0 means that every T patient has an outcome better than that for every C patient. AUC has been called “The Common Language Effect Size” ( McGraw and Wong 1992) or an “intuitive” effect size ( Acion et al, in press ), suggesting its relevance to interpreting clinical significance. Because AUC ranges from 0 to 1, to get the scaling of Figure 1, we can use 2AUC − 1.
What is Cohen's effect size?
When an RCT outcome measure is scaled, the most common effect size is Cohen’s d ( Cooper and Hedges 1994, Hedges and Olkin 1985 ), the difference between the T and C group means, divided by the within-group standard deviation. This effect size was designed for the situation in which the responses in T and C have normal distributions with equal standard deviations.
Is a RCT underpowered?
Then the RCT will typically be underpowered. In such studies, clinically significant results might well not be found statistically significant. In contrast, in an RCT powered correctly, it will seldom happen (less than 5% of the time) that T will be declared statistically significantly better than C when it is not, and it will seldom happen (far less than 20% [100%–80%] of the time) that a T that is clinically significantly better than C will not be declared statistically significantly better as well.
What is a nonsignificant test?
Conversely, a “nonsignificant” result means that the data are not sufficient to support a contention of nonrandomness— a comment on the quality of the data, not the quality of T versus C. It is a serious misinterpretation to suggest that a “nonsignificant” test comparing T and C indicates equivalent effects or to use terms such as “marginally significant,” “a trend toward significance,” and so on in reporting a nonstatistically significant result. These are merely different ways of saying that the study was not designed quite well enough to be able to establish a nonrandom difference between T and C by conventional standards ( p < .05). Asking for “post hoc” power calculations, too, is troublesome ( Levine and Ensome 2001, Tukey 1993 ). Preferable would be an effect size whose possible clinical significance could be evaluated and thus a judgment made as to whether pursuing the effect in future, better-designed RCTs remains warranted.
Why are P values miscalculated?
P values alone have problems. First, p values are often miscomputed, the result of misapplication of a test or of post hoc testing or multiple testing leading to exaggeration of significance. The two most obvious sources of miscomputation are possible but least likely: 1) errors in arithmetic; or 2) an intent to deceive; however, even in absence of errors, “naked” p values are not sufficient for an inference of clinical significance.
What is the gold standard method used to compare the effectiveness of different treatments or exposures?
Randomised controlled trials are the `gold standard’ method used to compare the effectiveness of different treatments or exposures since subjects are randomly assigned into different exposure groups rendering the two groups comparable for both known, and unknown, baseline confounders. Because of this comparability, the effect estimates obtained in RCTs can be interpreted as causal effects in that they provide an estimate of the effect of exposure on outcome that is unlikely to be explained by other factors such as confounding or reverse association. Once it is not possible to randomise, the parameter estimates obtained from an observational analysis are associational and may, or may not, have a causal interpretation. Methods have been developed that can disentangle association from causation in an observational setting but these require strong assumptions and can be very sensitive to violations of these assumptions.
What is an IV analysis?
An IV analysis addresses the case where there are some confounders that are either unknown or unmeasured. For exposure X and outcome Y, let U represent the set of unmeasured factors confounding the association between X and Y. For two variables A and B, the notation A ⊥ B denotes that A is independent of B. For a variable Z to be an IV it needs to satisfy the following three conditions:
What is the bias of 2SLS IV?
The unadjusted 2SLS IV model was biased at small sample sizes with fairly high variability (SD ≥ 2.50) across the effect estimates . The uncertainty in the effect estimates led to large bias and very low power to detect a statistically significant treatment effect at small sample sizes ( N = 2000). The bias and variability in the effect estimate reduces as the sample size increases leading to an increase in both the power and coverage of the effect estimates. Adjusting for measured covariates in the 2SLS IV model led to a large reduction in the variability of the effect estimates across all sample sizes and also makes the method more robust to misspecification of the first stage regression [ 28 ]. The bias of the effect estimates was also reduced, especially at small sample sizes, and power and coverage were both high for larger sample sizes ( N ≥ 20,000).
What is the purpose of unadjusted 2SLS IV models?
Unadjusted 2SLS IV models were fitted with robust standard errors [ 9] to give an estimate of the average causal treatment effect on the outcome. The first and second stage regression models are given in Eqs. 8 and 9 respectively:
What is an adjusted linear model?
An adjusted linear model was fitted to give a naïve estimate of the treatment effect. Under the strong and unverifiable assumption of `no unmeasured confounding’, this would be an estimate of the causal effect of treatment. The fitted linear model adjusted for all measured covariates is:
What is weak IV?
A weak IV is an instrument that does not explain much of the variability in the exposure X [ 14 ]. Different strengths of IV were assessed by varying the α1 parameter. The strength of unmeasured confounding of the treatment-outcome association on the results of the IV analysis was assessed by varying α2 and β5. The strength of causal treatment effect β1 was varied throughout the simulation study. An additional parameter β6 was introduced to (3) to assess the effect of a direct path between the IV and outcome (see Fig. 1 ). In this last scenario the outcome, was generated using (4):
What is IPTW in statistics?
The inverse probability of treatment weighting (IPTW) approach uses weights, obtained from the propensity score, so that the distribution of observed baseline covariates is independent of treatment assignment within the weighted sample [ 6 ]. Weighted regression models can then be used to obtain an estimate of the treatment effect. Propensity score stratification, whereby subjects are ranked according to their propensity score and then split into strata based on pre-defined thresholds [ 6] was also considered.
What is the difference between treatment effect and effect size?
The distinction between “Treatment effect” and “Effect size” lies not in the index but rather in the substance of the meta-analysis. When the meta-analysis looks at the relationship between two variables or the difference between two groups, its index can be called an “Effect size”. When the relationship or the grouping is based on a deliberate intervention, its index can also be called a “Treatment effect”.
What is treatment effect?
Meta-analysts working with medical studies often use the term “Treatment effect”, and this term is sometimes assumed to refer to odds ratios, risk ratios, or risk differences, which are common in medical meta-analyse s.
What characterizes an effect size?
What characterizes an effect size (and treatment effect) is that it looks at effects. Other meta-analyses do not look at effects but rather attempt to estimate the event rate or mean in one group at one time-point. For example, “What is the risk of Lyme disease in Wabash” or “What is the mean SAT score for all students in Utah”. These kinds of indices are called simply “Point estimates”, a generic category that includes the category Effect size, which in turn includes Treatment effects.
What is comprehensive meta analysis?
Comprehensive Meta-Analysis is a powerful computer program for meta-analysis. The program combines ease of use with a wide array of computational options and sophisticated graphics.
Why are effect sizes used in meta-analyses?
2. Unlike p-values, effect sizes can be used to quantitatively compare the results of different studies done in different settings. For this reason, effect sizes are often used in meta-analyses.
How to calculate effect size?
Using this formula, the effect size is easy to interpret: 1 A d of 1 indicates that the two group means differ by one standard deviation. 2 A d of 2 means that the group means differ by two standard deviations. 3 A d of 2.5 indicates that the two means differ by 2.5 standard deviations, and so on.
What is the mean score of a group 1?
The mean score for group 1 is 90.65 and the mean score for group 2 is 90.75. The standard deviation for sample 1 is 2.77 and the standard deviation for sample 2 is 2.78.
What are the advantages of effect sizes?
An effect size helps us get a better idea of how large the difference is between two groups or how strong the association is between two groups. A p-value can only tell us whether or not there is some significant difference or some significant association. 2.
What does larger effect size mean?
The larger the effect size, the larger the difference between the average individual in each group.
What does 0.3 mean in effect size?
Another way to interpret the effect size is as follows: An effect size of 0.3 means the score of the average person in group 2 is 0.3 standard deviations above the average person in group 1 and thus exceeds the scores of 62% of those in group 1.
What is effect size?
An effect size is a way to quantify the difference between two groups. While a p-value can tell us whether or not there is a statistically significant difference between two groups, an effect size can tell us how large this difference actually is. In practice, effect sizes are much more interesting and useful to know than p-values.
Why is it important to report effect sizes in research papers?
Increasing the sample size always makes it more likely to find a statistically significant effect, no matter how small the effect truly is in the real world. In contrast, effect sizes are independent of the sample size. Only the data is used to calculate effect sizes. That’s why it’s necessary to report effect sizes in research papers ...
What does a large effect size mean?
It indicates the practical significance of a research outcome. A large effect size means that a research finding has practical significance, while a small effect size indicates limited practical applications.
What would a measure of practical significance show?
Adding a measure of practical significance would show how promising this new intervention is relative to existing interventions.
What is statistical significance?
Statistical significance is denoted by p- values, whereas practical significance is represented by effect sizes. Statistical significance alone can be misleading because it’s influenced by the sample size. Increasing the sample size always makes it more likely to find a statistically significant effect, no matter how small ...
What does effect size mean in statistics?
Revised on February 18, 2021. Effect size tells you how meaningful the relationship between variables or the difference between groups is. It indicates the practical significance of a research outcome. A large effect size means that a research finding has practical ...
What are some examples of APA guidelines?
The APA guidelines require reporting of effect sizes and confidence intervals wherever possible. Example: Statistical significance vs practical significance. A large study compared two weight loss methods with 13,000 participants in a control intervention group and 13,000 participants in an experimental intervention group.
